A computer-aided diagnosis system using deep-learning analysis to detect lung lesion locations and quantitatively characterize the lesions on computed tomography (CT) images offered a fast and convenient approach for assisting radiologists in the diagnosis of lung nodule pathologies. These findings were presented by Liu et al during ASCO Breakthrough: A Global Summit for Oncology Innovators (Abstract 27).
Worldwide, among men and women combined, lung cancer is the most commonly diagnosed and deadly cancer, with approximately 2,093,876 new cases diagnosed in 2018 and 1,761,007 deaths reported. Although the early detection and treatment of lung cancer improves survival outcome, the accurate identification and characterization of small lung nodules is challenging.
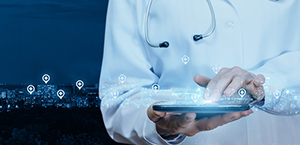
Photo credit: Getty
Study Methodology
The researchers employed deep-learning analysis for lesion detection in patients and performed image-processing techniques to generate quantitative morphology features for assisting lesion diagnosis. The data collected included 3,956 lung CT series (slice thickness ≤ 3 mm) with multiple lung nodules from 15 class A hospitals in China, 1,155 lung CT scans from the Luna16 datasets, as well as CT scans from the datasets of the Kaggle Data Science Bowl 2017. Lung nodule annotation was then performed by two experienced radiologists and further assessed by four senior associate chief physicians.
The obtained CT images were randomly selected and split to construct training, validation, and test datasets. After preprocessing, a pretrained ResNet-18 framework was transferred to develop a robust detection system to detect the possible lung lesion locations with corresponding probabilities.
KEY POINTS
- A computer-aided diagnosis system was able to accurately detect lung lesion locations and quantitatively characterize the detected lesions on CT images.
- The system’s image processing algorithm was able to generate diagnostic assisting features for each detected lesion, including longest diameter, shortest diameter, volume, largest cross section area, and density type: calcified, solid, partial solid, and ground-glass opacity.
Results
The researchers found that the resulting detection system yielded free-response receiver operating characteristic of 0.4663, recall of 82.46%, and precision of 36.06% for 5-mm to 30-mm nodules. Each detected lesion was labeled by its bounding box and was then analyzed through an image-processing algorithm to generate diagnostic assisting features, including longest diameter, shortest diameter, volume, and largest cross-section area as well as its density type (calcified, solid, partial solid, and ground-glass opacity).
“The proposed computer-aided diagnosis system offers a fast and convenient approach for assisting the diagnosis of lung nodule pathologies, and it is beneficial to relate our research to the current framework of lung cancer diagnosis,” concluded the study authors.
Disclosure: Funding for this study was provided by Linkdoc Technology. For full disclosures of the study authors, visit coi.asco.org.