A study investigating a computational method that integrates deep learning on histology specimens with clinicodemographic variables has found that the artificial intelligence may help predict response to immune checkpoint blockade among patients with advanced melanoma. This computational approach has the potential for integration into clinical practice; could assist oncologists in identifying patients who are at high vs low risk for disease progression while on immunotherapy; and could help to minimize treatment toxicity. The study by Johannet et al was published in Clinical Cancer Research.
Although immune checkpoint blockade has revolutionized the treatment for patients with advanced melanoma and other cancers, only a fraction of patients benefit from the therapy, highlighting the need to find approaches to identify patients who are most likely to respond.
Study Methodology
The researchers used data from a training cohort of 121 patients with metastatic melanoma treated with immune checkpoint blockade between 2004 and 2018. All of the patients were treated with first-line anti–CTLA-4 therapy, anti–PD-1 therapy, or a combination of both, and clinical outcomes were recorded as disease progression or response (which included complete and partial responses). Patients with stable disease were excluded for this proof-of-principle study.
The researchers used deep convolutional neural networks to analyze digital images of metastatic melanoma tumors and identify patterns associated with treatment response. They then developed a response classifier that aimed to predict if a patient’s untreated tumor would respond to immune checkpoint blockade or progress following treatment. The deep convolutional neural networks response classifier was validated in an independent cohort of 30 patients with metastatic melanoma treated at Vanderbilt-Ingram Cancer Center between 2010 and 2017.
Kaplan-Meier curves were used to compare progression-free survival between the groups. The classifier was validated on two slide scanners: Aperio AT2 and Leica SCN400.
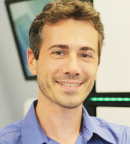
“There is potential for using computer algorithms to analyze histology images and predict treatment response…, but more work needs to be done using training and testing datasets, along with additional validation parameters, in order to determine whether an algorithm can be developed that achieves clinical-grade performance and is broadly generalizable."— Aristotelis Tsirigos, PhD
Tweet this quote
Results
The multivariable classifier predicted response with the area under the curve (AUC) 0.800 on images from the Aperio AT2 and AUC 0.805 on images from the Leica SCN400. The classifier accurately stratified patients into high- vs low-risk groups for disease progression. The patients from Vanderbilt-Ingram Cancer Center classified at high risk for disease progression had significantly worse progression-free survival than those classified at low risk (P = .02 for the Aperio AT2; P = .03 for the Leica SCN400).
Translational Relevance
“Histology slides and patients’ clinicodemographic characteristics are readily available through standard of care and have the potential to predict immune checkpoint inhibitor treatment outcomes. With prospective validation, we believe our approach has potential for integration into clinical practice,” concluded the study authors.
“There is potential for using computer algorithms to analyze histology images and predict treatment response…, but more work needs to be done using training and testing datasets, along with additional validation parameters, in order to determine whether an algorithm can be developed that achieves clinical-grade performance and is broadly generalizable,” said study author Aristotelis Tsirigos, PhD, Professor in the Institute for Computational Medicine at NYU Grossman School of Medicine, in a statement.
Disclosure: Funding for this study was provided by NYU Melanoma Specialized Program of Research Excellence grant, the Melanoma Research Alliance, the American Cancer Society, the Laura and Isaac Perlmutter Cancer Center, and the Onassis Foundation. For full disclosures of the study authors, visit clincancerres.aacrjournals.org.