An ensemble of machine-learning algorithms could help improve the accuracy of breast cancer screenings when used in combination with assessments from radiologists, according to a study published by Schaffter et al in JAMA Network Open.
The study was based on results from the Digital Mammography (DM) DREAM Challenge, a crowd-sourced competition to engage an international scientific community to assess whether artificial intelligence (AI) algorithms could meet or beat radiologist interpretive accuracy.
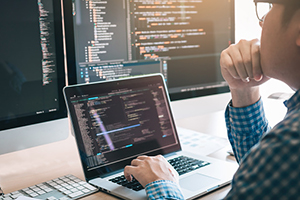
Photo credit: Getty
“Based on our findings, adding AI to radiologists’ interpretation could potentially prevent 500,000 unnecessary diagnostic workups each year in the United States. Robust clinical validation is necessary, however, before any AI algorithm can be adopted broadly,” said Christoph Lee, MD, MS, Professor of Radiology at the University of Washington School of Medicine and physician at the Seattle Cancer Care Alliance; he was the lead radiologist for the Challenge and co–first author of the paper.
Algorithm Details
Algorithms used solely images (challenge 1) or used a combination of images, previous examinations (if available), and clinical and demographic risk factor data (challenge 2). The algorithms then output a score that translated to yes/no for cancer within 12 months. An ensemble method—which aggregated the top-performing algorithms and radiologists’ recall assessment—was developed and then tested.
A total of 144,231 screening mammograms from 85,580 women in the United States, 952 of which were positive for cancer ≤ 12 months from screening, were used to train the machine-learning algorithms. A second validation cohort included 166,578 examinations from 68,008 women in Sweden, 780 of whom were positive for cancer.
Results
The best algorithm achieved an area under the curve of 0.858 for the women in the United States and 0.903 for the women in Sweden, and 66.2% (U.S.) and 98.5% (Sweden) specificity at the radiologists’ sensitivity, which was lower than radiologists practicing in the community setting.
While no single algorithm outperformed radiologists, a combination of the best-performing algorithms plus radiologists' assessments improved screenings' overall accuracy—bringing the area under the curve to 0.942 and an improved specificity—92%—at the same sensitivity.
Gustavo Stolovitzky, Director of the IBM Translational Systems Biology and Nanobiotechnology Program and Founder of the DREAM Challenge, added, “Our study suggests that an algorithmic combination of AI and radiologist interpretations could provide a mechanism for significantly reducing unnecessary diagnostic workups in the United States alone.”
Disclosure: The researchers’ funding included the National Cancer Institute and American Cancer Society. For full disclosures of the study authors, visit jamanetwork.com.