As reported in the Journal of Clinical Oncology, Aziz Nazha, MD, and colleagues have developed a model for predicting the risk of disease progression for individual patients with myelodysplastic syndromes (MDS).
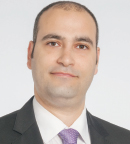
Aziz Nazha, MD
To develop the prediction model for overall survival and leukemic transformation, comprehensive clinical and molecular data from a training cohort of 1,471 patients with MDS were analyzed using machine-learning techniques. A random survival algorithm was used to generate the model, with the final model being tested in an external validation cohort and several additional cohorts.
Key Findings
The final model in the training cohort consisted of:
- Chromosomal karyotype
- Platelet count
- Hemoglobin level
- Bone marrow blast percentage
- Age
- Absolute lymphocyte count
- Absolute neutrophil count
- World Health Organization 2016 MDS classification
- White blood cell count
- Absolute monocyte count
- Peripheral blast percentage
- Seven individual gene mutations (RUNX1, RAD21, SRSF2, SF3B1, STAG2, ASXL1, and TP53)
- Mutation number.
Internal cross-validation of the final model showed c-index scores of 0.74 (95% confidence interval [CI] = 0.73–0.75) for overall survival and 0.81 (95% CI = 0.80–0.82) for leukemia transformation in the training cohort. In an independent validation cohort of 465 patients, c-index scores for the final model were 0.71 (95% CI = 0.73–0.75) for overall survival and 0.84 (95% CI = 0.73–0.75) for leukemia transformation; in comparison, c-index scores for overall survival were 0.66 (95% CI = 0.62–0.67) using the International Prognostic Scoring System (IPSS) and 0.67 (95% CI = 0.62–0.68) using the IPSS-Revised.
In an additional cohort consisting of 75 patients with higher-risk MDS from a North American clinical trial, the model yielded a c-index of 0.68, compared with 0.57 for IPSS. The model was also validated in a cohort of patients with paired samples at different time points during disease course and a cohort of patients who underwent hematopoietic stem cell transplantation.
The model, titled Personalized Prediction Model for Myelodysplastic Syndromes, is available for use online.
The investigators concluded, “A personalized prediction model on the basis of clinical and genomic data outperformed established prognostic models in MDS. The new model was dynamic, predicting survival and leukemia transformation probabilities at different time points that are unique for a given patient, and can upstage and downstage patients into more appropriate risk categories.”
Dr. Nazha, of the Cleveland Clinic Center for Clinical Artificial Intelligence, Taussig Cancer Institute, Cleveland Clinic, is the corresponding author for the Journal of Clinical Oncology article.