Use of a multimodal deep learning–based model led to more accurate and earlier identifications of cancer cachexia than standard clinical and radiological observations, according to findings presented at the 2025 American Association for Cancer Research (AACR) Annual Meeting (Abstract 1143).
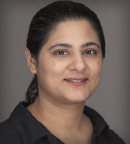
Sabeen Ahmed
“Compared with traditional biomarkers, AI-driven biomarkers may enable more sensitive and accurate detection of cancer cachexia by uncovering complex patterns missed by traditional analyses,” said lead study author Sabeen Ahmed, a graduate student at the University of South Florida and Moffitt Cancer Center.
When comparing against manual expert analysis, the model’s estimates of skeletal muscle area in computed tomography (CT) scans differed by a median of 2.48% in patients with pancreatic, colorectal, and ovarian cancers.
“The median discrepancy of 2.48% indicates that, on average, the model’s measurements of skeletal muscle were very close to the expert radiologists’ measurements, demonstrating the high reliability of our AI-based approach,” Ms. Ahmed explained.
Study Methods and Results
Physician-led detection of cancer cachexia is reliant upon the monitoring of skeletal muscle area on CT scans and manually annotating changes, which can be labor-intensive and often makes identification of cachexia occur too late in the progression of the disease.
To find a more efficient and reliable approach to identifying cancer cachexia, the researchers developed a multimodal, AI-driven multilayer perceptron model that integrates information from imaging scans and multiple types of routine clinical data. They trained two deep-learning models (nnU-Net 2D) with annotated CT scans from 50 patients with gastroesophageal cancer and 15 with pancreatic cancer and established uncertainty estimation methods and post-hoc calibration. The model was made to flag skeletal muscle area estimations that had a possibility for error so that the scans could be reviewed by experts.
In the gastroesophageal dataset, the model achieved an average Dice score, or measurement of similarity between the prediction and truth, of 97.8% for skeletal muscle segmentation.
Strong correlations were found in skeletal muscle area estimation differences between the model’s analysis and expert review, with a coefficient of 0.83 for variance, 0.76 for entropy, and 0.73 for the coefficient of variation. The model achieved 70% accuracy for cachexia prediction with an F1 machine-learning score for accuracy of 76.92%.
In terms of analysis for the patient’s survival, the model showed greater accuracy than clinical data alone by a difference of 5.6% in the pancreatic cancer dataset, 5.9% in the colorectal cancer dataset, and 1.5% in the ovarian cancer dataset.
“Our AI-driven multimodal approach provides a scalable and objective solution for detecting cancer cachexia using multiple types of data collected at the time of cancer diagnosis, potentially allowing health-care providers to initiate interventions to mitigate cachexia earlier in the disease course,” Ms. Ahmed stated. “The findings highlight the growing potential of machine learning to revolutionize cancer care and enable personalized treatment plans.”
The study authors noted that the model has not been trained and validated for use in other cancer types yet, nor has it been trained for using other forms of imaging scans beyond CT scans, which limits current use of the model. Additionally, missing or noisy clinical and imaging data could impact real-world applications of the model.
Disclosure: For full disclosures of the study authors, visit aacr.org.