Identifying patients at risk for adverse outcomes and intervening with intensive clinical services may improve cancer care while saving billions of dollars in avoidable emergency department (ED) visits. And, in fact, cancer centers may already have all the information they need to do so, according to research presented during the 2020 ASCO Quality Care Symposium.1,2
Findings from a pair of quality improvement initiatives showed that analysis of internal data and system patterns helped to identify patients with cancer who may benefit from further support. In the case of one cancer center, data-driven changes to practice patterns also led to significant decreases in inpatient admissions and ED visits.
“Based on changes in workflow along with adjustments in provider schedules and access for patients, we’ve aligned our institution with better at-home side effect management while relieving some of the burden to patients during their difficult time of outpatient chemotherapy,” said Anne M. Barry-Weers, BS, BSN, MS, Quality Director, System Cancer Service Line, Advocate Aurora Health, Milwaukee. “As one might expect, as admissions decrease, treatment costs to both the patient and the institution are impacted as well.”
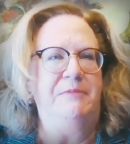
“We’ve aligned our institution with better at-home side effect management while relieving some of the burden to patients during their difficult time of outpatient chemotherapy.”— Anne M. Barry-Weers, BS, BSN, MS
Tweet this quote
Addressing Gaps in Patient Care
As Ms. Barry-Weers explained, the increasing number of patients receiving chemotherapy in a hospital outpatient department has led to gaps in care. This places a burden on patients who may have to manage the delayed onset of chemotherapy side effects at home or have limited access to providers in the outpatient setting who can best manage their care. These gaps are also exacerbated by poor communication.
“Patients often assume that little can be done about their symptoms, which leads them not to seek outpatient medical assistance and allows those symptoms to worsen,” stated Ms. Barry-Weers.
To address these issues, Ms. Barry-Weers and colleagues used the electronic health record to capture specific data points, including the reason for the visit, the time of day, the day of week, and the cancer type. This population included all payer patients with an ED visit or inpatient admission within 30 days of receiving chemotherapy.
After analysis revealed that the greatest percentage of patients were coming to the hospital Monday through Friday between 8:00 AM and 4:00 PM, a subcommittee of system operational leaders brainstormed and initiated several processes. They included enhancing patient triage, restructuring workflow, introducing the “Call Us First Campaign” education initiative, re-evaluating clinic access for pain management medications, and distributing free thermometers to patients allowing accurate assessment of symptoms while at home.
Improvement in Total Admissions
KEY POINTS
- Performance improvement strategies have demonstrated an impact on decreasing both inpatient admissions and emergency department visits by patients with cancer.
- A clinical tool developed with machine learning showed the potential to identify high-risk patients with cancer and to reduce unplanned care events.
A study of all outpatient chemotherapy infusions in the fourth quarter of 2018 and the first quarter of 2020, totaling 2,018 and 2,064 infusions, respectively, showed significant improvement in total admissions. Implementation of the initiatives resulted in a decrease in both ED and inpatient admissions. The latter fell from 10.7% to 8.3%, whereas visits to the emergency department (patients who were treated and released) decreased from 5.8% to 4.2%.
According to Ms. Barry-Weers, open clinic hours (8:00 AM to 4:00 PM) was the time of day most impacted by these initiatives. The percentage of patients admitted for pain as a primary diagnosis also decreased significantly from 5.2% in 2018 to 3.6% in 2020.
While still evolving, these initiatives have continued to demonstrate an impact on decreasing both inpatient admissions and ED visits, Ms. Barry-Weers concluded.
Machine-Learning Tool Identifies High-Risk Patients
A separate strategy leveraging machine-learning offers another approach to reducing unplanned care events, according to Lorinda Coombs, PhD, FNP, AOCNP, BC, of the Huntsman Cancer Institute, University of Utah, Salt Lake City.
As Dr. Coombs explained, a collaboration with Flatiron Health identified the potential role of a machine-learning clinical tool to identify patients who might be at risk for a visit to the emergency department or who would be eligible for enrollment into Huntsman at Home, an Oncology Hospital at Home model that provides acute hospital-level care by nurse practitioners and registered nurses in the patient’s home. The machine-learning tool took structured data from the electronic health record, including laboratory values, albumin levels, liver function tests, and visit history, and produced a list of high-risk patients delivered to clinicians weekly.
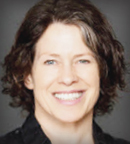
Lorinda Coombs, PhD, FNP, AOCNP, BC
Following retrospective validation, Dr. Coombs and colleagues designed a prospective study to analyze the clinical utility of the tool. Patients with cancer who received care at Huntsman Cancer Institute between January 4 and February 7, 2020, were included in the analysis and were randomly assigned to two prospective cohorts. The first cohort was used to identify patients who were eligible for Huntsman at Home services and was assessed by a clinical reviewer. The second cohort was used to identify patients as having a high or low risk a visit to the emergency department within 60 days. Patients were followed for 60 days to assess the precision of the outcome forecast.
High-Risk Patients More Likely to Visit Emergency Department
As Dr. Coombs reported, a total of 1,236 patients were analyzed in the model (median age, 65; 53% were female; 84% were White). The most common cancers were breast, prostate, lung, and myeloma, and the observed prevalence of a visit to the emergency department within 60 days was 7%.
“Patients who were identified as high risk by the tool were 5.4 times more likely to visit the emergency department within 60 days than those identified as low risk,” said Dr. Coombs. She noted that the tool classified 9% of patients as high risk.
Dr. Coombs acknowledged several limitations to the study. Within the context of oncology care, short-term follow-up is 60 days, but even patients with advanced metastatic disease can live for several years, she said. In addition, information received about ED visits was limited to in-network data, so some admissions may have been missed. Dr. Coombs also highlighted the lack of racial and ethnic diversity in the study. Finally, she noted, the pandemic may have influenced results if patients avoided the emergency department to prevent exposure to COVID-19.
Dr. Coombs and colleagues are planning a longer-term follow-up to assess the stability of the model over time, with a more diverse patient population. The researchers would also like to expand the tool to include other clinical outcomes, such as inpatient admissions and overall survival.
DISCLOSURE: Ms. Barry-Weers reported no conflicts of interest. Dr. Coombs reported financial relationships with Alexion Pharmaceuticals and Sanofi.
REFERENCES
1. Barry-Weers AM, Huibregtse C, Mitchell D, et al: Leveraging performance improvement strategies to decrease emergency department visits and inpatient admissions of patients receiving IV chemotherapy. 2020 ASCO Quality Care Symposium. Abstract 11.
2. Coombs LA, Orlando A, Adamson BJS, et al: Prospective validation of a clinical tool developed with machine learning to identify high-risk patients with cancer and reduce emergency department visits. 2020 ASCO Quality Care Symposium. Abstract 254.