The ASCO20 Virtual Scientific Program was different in many ways, not only because of the virtual modality forced by the COVID-19 pandemic, but also because of the resilience of the scientific society and my colleagues around the world.
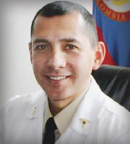
Luis Eduardo Pino Villarreal, MD, MSc, MBA
I’m in the plenary session of the ASCO 2025 Annual Meeting. The second trial to present is a prospective validation of a deep-learning algorithm that was trained using a genomic dendrogram and analyzed through quantic computation to improve directed therapies and outcomes in all stages of non–small cell lung cancer. The design of this trial was agile, with a response-adaptive randomization. Patients in continued response were linked to an intelligent platform for follow-up using molecular response by liquid biopsy dynamics. This platform allowed capture of adverse events; patients and physicians reported outcomes also. The trial was positive for primary and secondary outcomes. I’m still clapping mentally.
This year, I decided to carry out a search of the abstracts presented during the meeting related to what I have called next-generation oncology: those developments in oncology leveraged by digital and cognitive technologies 4.0 (artificial intelligence [AI], machine learning, deep learning, Internet of things, and digital platforms). I identified 272 papers, mainly in abstract format. After reviewing them, I chose 13 works among abstracts, posters, and oral presentations to share as a compilation of next-generation oncology highlights.
Humankind and Machine
An INCREDIBLE discussion by Sanjay Aneja, MD, of the Department of Therapeutic Radiology at Yale School of Medicine, addressed whether AI is ready for clinical practice.1 Dr. Aneja’s discussion was a much-needed tour of AI’s strengths and limitations for its implementation and development in the clinical world.
I think the main limitations of AI in the clinical scenario are the high heterogeneity of data sources, which increases the risk of bias, and the definition of ideal interventions to change how to measure prospectively changes leveraged by AI in the next generation of oncology. Dr. Aneja’s last message is to create validation and implementation of AI and machine-learning solutions, because the data sets are getting larger and more robust. Thus, natural intelligence and traditional analytic methods will be clearly insufficient. My last comment is related to the discussion title; instead of Humankind Versus Machine I think it could be Humankind and Machine.
“Data sets are getting larger and more robust, so natural intelligence and traditional analytic methods will be clearly insufficient.”— Luis Eduardo Pino Villarreal, MD, MSc, MBA
Tweet this quote
Robotics and Cognitive Automation
Khammad et al focused on the application of machine-learning algorithms for the diagnosis of primary brain tumors.2 This is a very interesting trial that was presented as an oral abstract and centers on a hospital in Uruguay. They designed and validated a diagnostic workflow with the implementation of modern machine-learning/AI approaches in a population model. This algorithm was based in R software, and they called it “La Tabla.” The network included clinical and histopathologic variables and was trained with three decision-tree algorithms. This trial reported predicted diagnoses using just clinical and histologic findings, with 94% and 88% accuracy, respectively. This could improve not only diagnosis, but also may serve as a reliable tool for second opinions in clinical neuro-oncology.
Cognitive Insights
Besse et al created and trained an algorithm for predicting molecular testing based on the metastatic pattern for stage IV lung adenocarcinoma.3 They did not report the accuracy of the algorithm, but it seems to have good clinical and molecular correlation for EGFR, ALK, and BRAF mutations. Free online access to the algorithm is now available after registration at http//tactic-ct.fr. Continual learning from new cases entered in the database will increase the sensitivity of this algorithm. This tool might save time, tumor tissue, and economic resources as well as accelerate access to personalized treatment.
Ma et al reported a real-world lung cancer cohort (5,003 patients), with clinical information extracted from electronic medical records of patients with lung cancer using various techniques of natural language processing and AI; they developed a system to automatically abstract outcomes.4 Images, lines of treatment, and biopsies were also abstracted as attributes of outcomes. This automated abstraction system improved human abstractor efficiency to curate outcomes, reducing curation time per patient by 90%. This is one of the few studies in which natural language processing has been successful.
Raghu et al developed a convolutional neural network (CXR-LC) from chest radiograph images and clinical variables in 41,856 persons between the ages of 55 and 74 to detect risk related to lung cancer.5 CXR-LC risk groups were associated with incident lung cancer in PLCO test data set smokers (very high vs low risk: 12.4 vs 1.1 lung cancers/1,000 person-years) with external testing in the NLST (all Centers for Medicare & Medicaid Services–eligible: 12.7 vs 2.3). The most interesting fact in this trial is that, for low-resource settings, this neural network may help to improve lung cancer detection. Now, we have some algorithms for lung nodules using tomography (even approved by the U.S. Food and Drug Administration [FDA]).
Sidey-Gibbons et al retrospectively surveyed 611 patients who had undergone breast cancer therapy at MD Anderson Cancer Center to assess the financial impact of their care.6 For this goal, they trained and validated a neural network, LASSO-regularized linear model, and support vector machines using data from the FACT-COST (focused average cost tracking) patient-reported outcome measures, alongside other financial indicators including income and insurance status. The algorithm predicted significant financial burden relating to cancer treatment with high accuracy (0.83; area under the ROC curve = 0.82, sensitivity = 0.81, specificity = 0.82). Key clinical predictors of financial burden from linear models were neoadjuvant therapy (β-regularized 0.12) and autologous, rather than implant-based, reconstruction (β-regularized 0.10). The algorithm showed accuracy in predicting an infrequently measured outcome related to breast cancer care.
Cognitive Engagement
Using secure digital messaging to proactively manage symptoms in patients on capecitabine, Sohal et al demonstrated greater medication adherence in patients on capecitabine, even with adverse events.7 Proactive symptom tracking supported the early identification of potential adverse events and effective nurse care coordination. They demonstrated a good outcome with a basic digital intervention.
Bowen et al implemented an automated program called Deep Lens VIPER, accessing the electronic medical records and pathology systems to effectively identify patients who are candidates for 20 ongoing recruiting clinical studies, probably using natural language processing assisted by human assistants.8 The final result was increased monthly candidate patient catchment for 16 of the 20 studies under investigation. This would result in approximately 600 patients annually added for final triage for studies being conducted.
Morgan et al used a chatbot and video education to engage high-risk people with BRCA mutations to improve genetic testing.9 As of the 12-week survey, 65.4% of these patients planned to proceed with recommended screening or scheduled risk-reducing surgery; 3.5% of those with negative genetic testing results and high-risk family histories reported undergoing further genetic testing. Satisfaction was high (mean = 9.58/10, standard deviation = 1.12) and unrelated to results (P > .05).
Shen et al focused on the feasibility of a digital medicine program in optimizing opioid pain control in patients with cancer (SWOG S1916).10 They described a digital medicine program called Proteus Discover consisting of an FDA-approved ingestible sensor made of dietary minerals co-encapsulated with patients’ medications, a wearable sensor patch, and a mobile device app. The app enabled patients to electronically transmit their medication adherence patterns to monitor opioid use in the treatment of metastatic cancer pain. This trial is very interesting and currently recruiting (ClinicalTrials.gov identifier NCT04194528).
Dias et al contributed to the field by asking patients about their preferences related to the use of digital technology with smartphones and wearables as well as telehealth.11 They attempted to identify motivational factors that might drive real-world study compliance for daily symptom reporting. The most motivating factor was access to health information that may help manage their symptoms on a daily basis (92%). Subsequent factors of interest included in-app reminders (88%) and assurance that a doctor would review the reported data and contact them to discuss reported symptoms (94%). This is good information to improve and better define the way we deliver information through digital technologies for patients.
Using a bidirectional digital health platform, Alford et al built a Health Insurance Portability and Accountability Act–compliant role-based digital health platform to support providers in patient engagement and care coordination in India.12 Nearly 200 patients with cancer participated in the pilot study. Patients’ medical records were digitized by oncology nurse navigators. The summarized longitudinal view of the patient’s medical history was used by oncologists for diagnosis and treatment planning. Participating clinicians noted the digital case summary saved time and effort in treatment planning. This platform also improved collaboration among nursing staff, medical oncologists, surgical oncologists, and radiation oncologists. I am looking forward to seeing changes in the oncology process indicators in a new report.
To address the barriers to early cancer detection in underserved communities, Munoz-Zuluaga et al designed a Spanish language app using educational, evaluative, and risk-factor questions.13 After the questions, brief explanations were provided to assess the population’s knowledge, educate users on breast and cervical cancers, and identify those in need of screening in Cali, Colombia. In addition to the identification of misconceptions, they found 31.5% of women (329 patients) were at risk and needed a mammogram and/or Pap smear. So far, 30% of women (98 patients) completed their recommended screening test(s). Barriers to enrollment in these programs included patient unwillingness, the use of fake contact information, limited available appointments, and denied access due to health-care coverage. This is an example of intelligent induction to screening, although sadly it demonstrates that access failures in health-care systems may be superior to digital improvements.
Great work! I apologize to authors of other studies that deserved to be included here. Next-generation oncology will continue to grow safely at the ASCO 2021 Annual Meeting.
DISCLOSURE: Dr. Pino Villarreal has had an advisory/consultant role with AstraZeneca, Roche, MSD, and BMS; and has served as a speaker for AstraZeneca, Roche, and BMS.
REFERENCES
1. Anjela S: Man versus machine: Is artificial intelligence ready for clinical practice? ASCO20 Virtual Scientific Program. Discussion of Abstracts 2009, 2010, and 2011.
2. Khammad V, Otero JJ, Izquierdo YC, et al: Application of machine learning algorithms for the diagnosis of primary brain tumors. ASCO20 Virtual Scientific Program. Abstract 2533.
3. Besse B, Dormieux A, Mezquita L, et al: Prediction of the molecular status in non-small cell lung cancer based on metastatic pattern: A free webtool powered by artificial intelligence. ASCO20 Virtual Scientific Program. Abstract 9535.
4. Ma M, Redfern A, Zhou X, et al: Automated abstraction of real-world clinical outcome in lung cancer: A natural processing and artificial intelligence approach from electronic health records. ASCO20 Virtual Scientific Program. Abstract e14062.
5. Raghu V, Mayrhofer T, Aerts H, et al: Deep learning to identify high-risk smokers for lung cancer screening from chest radiographs. ASCO20 Virtual Scientific Program. Abstract 1519.
6. Sidey-Gibbons C, Asaad M, Pfob A, et al: Machine learning algorithms to predict financial toxicity associated with breast cancer treatment. ASCO20 Virtual Scientific Program. Abstract 2047.
7. Sohal M, McLarty S, Friend KE, et al: Using digital engagement to proactively manage symptoms in patients on capecitabine. ASCO20 Virtual Scientific Program. Abstract 12079.
8. Bowen TJ, Stephens L, Vance M, et al: Novel artificial intelligence (AI)-based technology to improve oncology clinical trial fulfillment. ASCO20 Virtual Scientific Program. Abstract 2052.
9. Morgan K, Symecko H, Kamara D, et al: The BRCA founder outreach study: Initial results of a digital health model. ASCO20 Virtual Scientific Program. Abstract 2007.
10. Shen S, Vaidya R, Darke A, et al: Feasibility of a digital medicine program in optimizing opioid pain control in cancer patients (SWOG S1916). ASCO20 Virtual Scientific Program. Abstract TPS12126.
11. Dias N, Yamamoto R, Faulkner KG: Preferences and motivational factors for the use of digital technology in real world oncology studies. ASCO20 Virtual Scientific Program. Abstract e14119.
12. Alford SH, Mahatma S, Mahatma R, et al: Using a bi-directional digital health platform for cancer care coordination and monitoring to enhance the cancer care continuum between patients, clinicians, and caregivers. ASCO20 Virtual Scientific Program. Abstract e14105.
13. Munoz-Zuluaga CA, Gallo-Perez JD, Perez-Bustos A, et al: Mobile apps: Breaking barriers to early cancer detection in underserved communities. ASCO20 Virtual Scientific Program. Abstract 2065.