Although most patients with terminal cancer, 87%, have end-of-life conversations with clinicians about their goals and preferences for care, on average, these discussions happen just 1 month before death and most often occur in acute care settings with clinicians who are not their treating oncologists.1
Studies show that early discussions about end-of-life care goals often result in patients receiving care in accordance with their wishes, feeling less distress, and experiencing a better quality of life.2 Despite these benefits, 71% of patients with advanced cancer reported that although they would want their physician to tell them how long they had left to live, only 18% said their oncologist provided them with a prognostic estimate.3
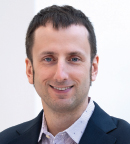
“Although the aim of this study was to increase the number of serious illness conversations between physician and patient, what we really want to do is improve the care patients receive at the end of their lives.”— Christopher R. Manz, MD
Tweet this quote
There are myriad reasons why serious illness conversations are not taking place earlier in the course of care—or at all—for patients with late-stage cancer, including patients’ reticence about raising end-of-life preferences and oncologists’ concern that initiating these conversations may increase anxiety and undermine patients’ hope. Another key reason is that oncologists’ prognostic estimates are often inaccurate. According to a large review of patients with advanced cancer with a median survival of 29 days, just 61% of physicians accurately estimated survival to within 4 weeks.3
Ensuring Patients Receive the End-of-Life Care They Want
The results from two recent studies by Christopher R. Manz, MD, Instructor at Harvard Medical School, and his colleagues may help to increase both the accuracy of prognostic predictions to aid in physician and patient treatment decision-making and the frequency of clinicians’ serious illness conversations with patients. The first study investigated whether a machine learning algorithm could accurately identify patients at risk of 180-day mortality, and the second study tested combining machine learning mortality predictions with behavioral nudges to clinicians to initiate serious illness conversations. The findings showed the technology accurately predicted short-term mortality risk for patients who outperformed traditional prognostic indices4 and, when combined with behavioral nudges to oncologists, increased clinician serious illness conversations by threefold over 16 weeks.5
The prognostic study included a cohort of 24,582 patients with a variety of cancers being treated within the University of Pennsylvania Health System during a baseline period between March 1, 2019, and April 30, 2019. The researchers used a retrospectively validated gradient-boosting machine learning algorithm embedded in oncology practices’ medical health record systems to predict 180-day mortality risk between 4 and 8 days prior to each patient’s oncology visit. The findings showed the algorithm accurately identified the risk of 180-day mortality in the high-risk group, with good discrimination and positive predictive value of 45.2% vs 3.1% in the low-risk group. When added to performance status– and comorbidity-based classifiers, the algorithm favorably reclassified patients.
In the stepped-wedge cluster randomized clinical trial, which included 14,607 patients and tested the effect of delivering machine learning–based mortality estimates with behavioral nudges to oncologists to increase serious illness conversations, the researchers found the intervention increased these conversations from about 1% to 5% of all patient encounters and from about 4% to 15% of encounters with patients having high predicted-mortality risk.
The ASCO Post talked with Dr. Manz about how integrating artificial intelligence (AI)-based algorithms into electronic health records may improve care for patients with advanced cancer, especially those with life-limiting disease.
Comparing AI and Conventional Prognostic Indicators
Please talk about the benefit of using a machine learning–based algorithm to predict the short-term mortality risk of patients with cancer compared with traditional prognostic indicators, such as tumor size, age, and performance status.
Our main reason for developing this prediction model was to help patients who might benefit from a serious illness conversation, regardless of how long they may live. We know that oncologists accurately identify only 35% of patients with cancer who are likely to die within 6 months. We wanted to develop an automated, accurate prognostic system to identify those patients with a high risk of dying within 180 days to ensure that physicians have a serious illness conversation with them to inform treatment decision-making and advance care planning.
Our algorithm model is not meant to supplant clinicians’ perspectives on prognostication using conventional indicators, including comorbidities or performance status, but rather to serve as a tool to augment conventional prognostic classifiers.
Calculating the Benefits of Knowing Short-Term Mortality
How do patients benefit from knowing they may have a risk for dying of their cancer over the next 6 months?
We know from a randomized clinical trial that patients with advanced cancer who have serious illness conversations with their oncologists have significant reductions in emotional suffering. When patients have documented goals-of-care discussions with their oncologists, they are also more likely to receive the care they want, are less likely to receive chemotherapy in the last month of their lives, and are less likely to die in the hospital.
“Our algorithm model is not meant to supplant clinicians’ perspectives on prognostication using conventional indicators, including comorbidities or performance status, but rather to serve as a tool to augment conventional prognostic classifiers.”— Christopher R. Manz, MD
Tweet this quote
We also know that, despite these benefits, most patients are not having these conversations early enough—or at all—to change the care they receive at the end of their lives.
How often should these discussions happen over the course of a patient’s care?
ASCO guidelines recommend that clinicians should initiate conversations about patients’ end-of-life preferences early in the course of incurable disease and then periodically, depending on changes in disease status, including cancer progression, and a decline in a patient’s functional status.6
Unfortunately, we know that this isn’t happening often enough. When these conversations do happen, they usually concentrate on patients’ clinical status and not on their goals and wishes for end-of-life care. The clinical information, and not the patient’s care preference, is then documented in the patient’s electronic medical records. As a result, when the patient shows up in the emergency room or is hospitalized, the patient’s end-of-life care goals might not be known and followed.
Using Behavioral Nudges to Spark Serious Illness Conversations
In your stepped-wedge cluster randomized study investigating how AI can spark serious illness conversations between oncologists and their patients, you found the algorithm increased the rate of discussions from about 1% to 5% of all patient encounters and from about 4% to 15% of encounters with patients having high predicted-mortality risk.5 How were physicians prompted to have these conversations with patients; what were the behavioral nudges?
In this study, we sent 78 oncology clinicians, including physicians, nurse practitioners, and physician assistants, a list of up to 6 patients they were scheduled to see the following week who had the highest predicted risk of 6-month mortality based on our validated machine learning prognostic algorithm; we said these patients may benefit the most from a serious illness conversation. We were trying to change clinician behavior, so in addition to the prompt about the patients, we incorporated other behavioral nudges as well, including individual serious illness conversation performance feedback, such as the number of serious illness conversations a clinician had in the past month, and peer -comparisons.
GUEST EDITOR
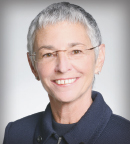
Jamie H. Von Roenn, MD, FASCO
Addressing the evolving needs of cancer survivors at various stages of their illness and care, Palliative Care in Oncology is guest edited by Jamie H. Von Roenn, MD, FASCO. Dr. Von Roenn is ASCO’s Vice President of Education, Science, and Professional Development.
The goal of this intervention was to help clinicians think every week about the patients who might benefit from these discussions. What we found is that the number of serious illness conversations spurred by these prompts increased, not just for the patients flagged as at highest risk in our algorithm, but for lower-risk patients who were not flagged by the algorithm as well. These results suggest that this type of intervention may help make serious illness conversations become a routine part of clinical practice.
Although the aim of this study was to increase the number of serious illness conversations between physician and patient, what we really want to do is improve the care patients receive at the end of their lives. Next, we want to see whether prompting more of these conversations actually results in improving end-of-life care. In this area, there is still more work to be done.
DISCLOSURE: Dr. Manz reported no conflicts of interest.
REFERENCES
1. Paladino J, Bernacki R, Neville BA, et al: Evaluating an intervention to improve communication between oncology clinicians and patients with life-limiting cancer. JAMA Oncol 5:801-809, 2019.
2. Bernacki R, Paladino J, Neville BA, et al: Effect of the serious illness care program in outpatient oncology. JAMA Intern Med 179:751-759, 2019.
3. Lambden J, Zhang B, Friedlander R, et al: Accuracy of oncologists’ life-expectancy estimates recalled by their advanced cancer patients: Correlates and outcomes. J Palliat Med 19:1296-1303, 2016.
4. Manz CR, Chen J, Liu M, et al: Validation of a machine learning algorithm to predict 180-day mortality for outpatients with cancer. JAMA Oncol 6:1723-1730, 2020.
5. Manz CR, Parikh RV, Small DS, et al: Effect of integrating machine learning mortality estimates with behavioral nudges to clinicians on serious illness conversations among patients with cancer: A stepped-wedge cluster randomized clinical trial. JAMA Oncol 6:e204759, 2020.
6. ASCO Guidelines: Patient-Clinician Communication Consensus Guideline. Available at www.asco.org/sites/new-www.asco.org/files/content-files/practice-and-guidelines/2017-patient-physician-comm-summary-table.pdf. Accessed March 31, 2021.