Immunotherapy with immune checkpoint inhibitors has made great strides in the treatment of many cancers, but only between 25% and 50% of patients respond with clinical benefit, and these agents come with adverse events and high price tags. Thus, preselection of patients who are likely to respond to this type of therapy is an important area of research.
Many studies have included tissue and blood samples from patients in the quest to identify valid biomarkers, with varying degrees of success. Markers such as PD-L1 expression and tumor mutational burden have shown some promise, but thus far, no biomarker has panned out as a universal way to identify patients who will and will not benefit from these important new agents.
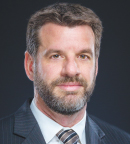
Matthew Schabath, PhD
Researchers at Moffitt Cancer Center, Tampa, Florida, may have some answers based on imaging characteristics if preliminary studies are borne out. These researchers used images of patients with non–small cell lung cancer (NSCLC) slated for treatment with immune checkpoint inhibitors to determine the likelihood of progression-free and overall survival.
Two studies presented during the 2020 American Association for Cancer Research (AACR) Virtual Annual Meeting II suggest that using radiomics—quantitative image analysis that extracts features from computed tomography (CT) scans or positron-emission tomography (PET) scans—may help guide treatment decisions related to immune checkpoint inhibitor therapy in patients with NSCLC.1,2
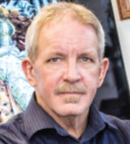
“Instead of just predicting whether a patient is going to do well, we can say which therapy is best.”— Robert Gillies, PhD
Tweet this quote
“It is very important to identify predictive biomarkers prior to treatment for help in treatment decision support. You don’t want to treat patients with a therapy they won’t respond to because this equates to lost time along with associated toxicities,” stated Matthew Schabath, PhD, of Moffitt Cancer Center, senior author of one of the studies and coauthor of another, along with Robert Gillies, PhD.
Validation of Clinical Radiomics Model
The first study analyzed CT scans of 332 patients with advanced NSCLC who were slated to undergo treatment with single- or double-agent immune checkpoint blockade.1 Using a combination of pretreatment radiomics and clinical data, they developed and validated a parsimonious clinical-radiomics model that could stratify patients into four risk groups based on the likelihood of poor survival: low, moderate, high, and very high. Notably, the very high–risk poor survival group had disease progression in less than 1 year and would likely benefit more from another therapeutic approach.
In this study, the most informative clinical variables were serum albumin and the number of metastatic sites. The most informative radiomics variable was grey level co-occurrence matrix inverse difference, which was an “Avatar” for a constellation of image features. Analyzing this Avatar feature in a “radiogenomics” data set identified it as being sensitive to tumor hypoxia, which is generally associated with immunosuppression and poor prognosis with a number of other therapies.
“The very high–risk group was associated with extremely poor outcomes: 0% survival at 3 years compared with 38.9% in the low-risk group. Similar findings were observed for progression-free survival: 0% at 3 years vs 29.8%, respectively,” said lead author Ilke Tunali, PhD, a postdoctoral fellow at Moffitt.
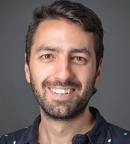
Ilke Tunali, PhD
“We found that those in the very high–risk group should not be treated with immunotherapy because they will not respond. We also found that our image-based biomarker was associated with specific gene expression related to tumor hypoxia and was associated with poor outcomes in patients with lung cancer even if they are not treated with immunotherapy,” Dr. Tunali reported. Further studies are needed to validate whether these image-based biomarker findings could apply to other therapies and other cancers, he added.
“The potential clinical application of this work is that pretreatment radiomics and clinical covariates can identify patients who are unlikely to respond and can eliminate unnecessary treatments,” Dr. Tunali said.
Deep Learning Software
The second study explored whether a score based on deep learning analysis of PET/CT scans can predict PD-L1 expression as well as a positive response to immune checkpoint
inhibitor therapy.2
“PD-L1 expression determined by immunohistochemistry is currently the only clinically approved biomarker to trigger treatment decisions,” said lead author of the study, Wei Mu, PhD, a postdoctoral fellow at Moffitt who developed the deep learning score for this study. “However, sampling bias and the dynamic nature of PD-L1 expression can affect the final results. Currently, the only way to determine PD-L1 expression is with an invasive biopsy, which can pose some risk for patients with lung cancer.”
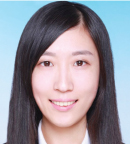
“The multiparametric radiomics signature could accurately predict durable clinical benefit in both the training and test cohorts.”— Wei Mu, PhD
Tweet this quote
The training and validation sets for using the deep learning score to predict PD-L1 status involved CT/PET scans from 400 patients with NSCLC. The deep learning score was found to be accurate in discriminating between patients with PD-L1–positive and –negative disease. Of note, this deep learning score was also validated to be predictive of response to immune therapy in an independent NSCLC cohort treated with immune checkpoint blockade.
For the second analysis, a multiparametric radiomics signature generated from radiomics features of PET/CT scans was used to predict progression-free and overall survival. Retrospective training and validation sets involved PET/CT scans from 146 patients with advanced NSCLC using the multiparametric radiomics signature to predict durable clinical benefit from immune checkpoint inhibitors. This approach was prospectively validated in 48 patients with advanced NSCLC being treated with immune checkpoint inhibitors.
“The multiparametric radiomics signature could accurately predict durable clinical benefit in both the training and test cohorts,” Dr. Mu said.
KEY POINTS
- Researchers have created an algorithm that relies on measurements already taken prior to treatment initiation to identify the likelihood of response to immune checkpoint inhibitors in patients with NSCLC prior to initiating treatment.
- This approach using PET/CT scan radiomics plus two clinical features may prove to be a reliable biomarker—one that does not require a biopsy.
- Since patients already undergo PET/CT scan prior to treatment and laboratory tests, this is a relatively noninvasive and inexpensive way to predict response.
Dr. Mu continued: “We have observed that, prior to therapy initiation, PET/CT fusion images can be used independently to predict PD-L1 status or durable clinical benefit in patients with advanced NSCLC. Both of these models can be leveraged to improve more precise and individualized decision support in the treatment of patients with advanced NSCLC.”
In addition to the study presented during the AACR Virtual Annual Meeting II, Dr. Mu and colleagues have used the deep learning score and conventional imaging to determine whether patients have an EGFR mutation and what their PD-L1 status is. This allows a decision between the two types of treatments without invasive biopsies.
Additional Commentary
“These two studies are exciting because they can be combined and, for truly the first time, we can generate a decision matrix between two therapies [immune therapy and anti-EGFR therapy] based on radiomics. Instead of just predicting whether a patient is going to do well, we can say which therapy is best,” Dr. Gillies said (he was involved in both studies).
Combining all these studies, Dr. Gillies stated: “A major challenge in the use of artificial intelligence for clinical decision support is the ‘black box’ nature of the scoring, which limits its clinical acceptance. We feel that these studies are both meaningful because they are adding biologic signatures [hypoxia, PD-L1, EGFR] to a machine-learned score, which we predict will increase their palatability and uptake.”
DISCLOSURE: Drs. Schabath, Tunali, and Mu reported no conflicts of interest. Dr. Gillies has received research support from HealthMyne.
REFERENCES
1. Tunali I, Tan Y, Gray JE, et al: Hypoxia-related radiomics predict checkpoint blockade immunotherapy response of non-small cell cancer lung patients. 2020 AACR Virtual Annual Meeting II. Abstract 5806.
2. Mu W, Tunali I, Gray JE, et al: Prediction of clinical benefits to checkpoint blockade in advanced NSCLC patients using radiomics of PET/CT images. 2020 AACR Virtual Annual Meeting II. Abstract 868.