Developed in 1925 by British statistician Sir Ronald Fisher, the P value is a measure that is ever-present in abstracts and studies, a small statistical tool that has enormous power to aid research being published in the literature or support drug approval. Over the past several years, however, a growing number of researchers have questioned whether the P value’s place in clinical trial findings should be reevaluated. To shed light on this issue, The ASCO Post spoke with epidemiologist and statistician Joshua Wallach, PhD, MS, Assistant Professor of Epidemiology at Yale School of Public Health.
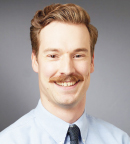
Joshua Wallach, PhD, MS
Self-Proclaimed ‘Meta-researcher’
Please tell the readers a bit about your background and current position/work.
I consider myself a “meta-researcher,” which means that most of my work focuses on studying how research is conducted and reported, how and when data should be synthesized, and how to improve research practices. I completed my PhD in epidemiology and clinical research at Stanford University, where I had the opportunity to work with the Meta-Research Innovation Center at Stanford (METRICS), a research center that focuses on evaluating and improving research reproducibility and transparency.
During my time with METRICS, I studied the status and trends of reproducibility and transparency across the biomedical literature, the use of P values, and the conduct of subgroup analyses in trials and meta-analyses. After completing my postdoctoral training at Yale, with the Collaboration for Research Integrity and Transparency, I joined the Department of Environmental Health Sciences at the Yale School of Public Health as Assistant Professor.
Currently, my research is divided across a number of overarching topics. For instance, this past year, I have been working on a number of projects focused on the quantity and quality of evidence generated for drugs and biologics after they are approved by the U.S. Food and Drug Administration.
Complexity of Defining P Values
Can you explain why the exact meaning of the P value and its implications are not well understood by many oncologists?
P values are ubiquitous across scientific fields. However, it is extremely difficult to succinctly and accurately translate what a P value means into something that is easy to understand.
When P values were first introduced by Sir Fisher, they were used as a way to determine the strength of evidence against a null/reference hypothesis. He used P values and the word “significant” to outline results that were supposed to be of interest and therefore should be analyzed in future evaluations. When P values were combined with formal hypothesis testing, things became a lot less intuitive.
P values are conditional probabilities, which means they depend on the assumptions someone makes for a study. A common, but simplified, technical definition of a P value is the probability of observing what you observed in your study, as well as more extreme (larger) results, if you assume that there is no effect or difference (null hypothesis) and that the statistical model used to compute the P value is correct.
“The P value is a conditional probability, which means that it depends on the assumptions someone makes for a study.”— Joshua Wallach, PhD, MS
Tweet this quote
Let us imagine a simplified hypothetical randomized experiment, where we are comparing a new and an existing cancer intervention. The outcome of interest is the difference between the two interventions. The null hypothesis would be that there is no difference between the interventions (that they are identical). We then conduct the study, calculate the difference between the groups, and try to understand how unlikely the observed outcome of the experiment would be, assuming there is no difference between the interventions.
This is where the P value comes in. It is a measure of the consistency between the trial data and the hypothesis being tested. If the study results in a P value less than 5%, it suggests that the observed results (or larger unobserved results) are unlikely if we assume our null hypothesis and all other assumptions used to conduct the test are correct. Studies typically use this as evidence to support their observed results over the null hypothesis. However, the reality is that a small P value indicates that the data are unusual given all the assumptions, and it is unclear which assumption, including the null hypothesis, is actually incorrect.
This example is drastically simplified, but it demonstrates why P values are difficult to define and not well understood by both researchers and the public. For instance, a P value of .05 does not mean there is a 5% chance that the null hypothesis is true. It also does not imply there is a 95% or greater chance that the null hypothesis is incorrect. When a P value is greater than 5%, it does not mean there is no difference between two interventions; it just means the observed result is statistically consistent with the selected null hypothesis.
Moreover, a P value does not measure whether the null hypothesis is false, since it is calculated assuming the null is true, and it does not tell you if something is actually right or wrong. P values also do not tell you anything about the magnitude of effect, the strength of evidence, the likelihood of something being replicated, or the probability that something is more or less likely to be a chance finding. When P values are reported alone in an abstract or full manuscript without effect estimates or measures of precision, they are essentially meaningless conditional probabilities, which are compared with an arbitrarily established cutoff at .05.
Closer Look at Use and Misuse of P Values
You and your colleagues conducted a study that looked at the use and misuse of P values. Could you share its design, objectives, and findings?
In 2016, I coauthored a paper with David Chavalarias, Alvin Li, and John Ioannidis published in JAMA where we looked at the reporting and evolution of P values across the biomedical literature between 1990 and 2015.1 Considering how widespread statistical testing methods are across scientific fields, as well as the growing concerns that P values are misunderstood, miscommunicated, and misused, we set out to determine some of the characteristics and trends in the literature. Briefly, the study had two main components: text-mining analyses of approximately 13 million abstracts and nearly 1 million full-text articles, and an in-depth manual assessment of 1,000 random abstracts.
There were a number of really interesting trends. We showed that more abstracts and articles were reporting P values over time and that almost all reported statistically significant analyses. We also found that P values were rarely reported with effect sizes and confidence intervals, which are needed to understand the magnitude and uncertainty of the outcome you are measuring. P values reported in abstracts were also lower (showing greater significance) than P values reported in full text, which could indicate selective reporting of significant findings in study abstracts. It was also interesting to observe how P values were often clustered around .05, which reflects widespread use of this cutoff in hypothesis testing.
“[In our study], we did not suggest that P values should be abandoned, but we noted that more stringent P value thresholds are likely necessary….”— Joshua Wallach, PhD, MS
Tweet this quote
Although our results could represent that findings are becoming more significant on average, they may also reflect an increasing number of variables and analyses, as well as bigger sample sizes. However, it is also possible that these findings simply reflect the pressure to publish significant results and how more analyses are being tested, which increases the chances of random findings. In our study, we did not suggest that P values should be abandoned, but we noted that more stringent P value thresholds are likely necessary, and alternative statistics should be helpful, as well as improved transparency and reporting of effect measures and uncertainty.
How Hypotheses Are Tested
In 2005, Dr. Ioannidis published an article titled “Why Most Published Research Findings Are False.”2 Can you comment on this bold statement?
It is great that this paper is still being discussed, nearly 15 years after it was first published. Since the publication of his article, there has been a growing interest in research reproducibility and transparency, and there have been a number of high-impact papers demonstrating high rates of nonreplication (eg, in psychology and cancer biology). Although the title of the article is certainly bold, it serves as an important reminder of how hypotheses are tested, how study biases can influence research, and that a study cannot tell us with certainty whether a research finding is actually “true”. This does not mean that research should not be conducted, but it highlights limitations of judging individual findings based on P values < .05. Individual findings need to be corroborated, and evidence needs to be accumulated over time.
In our P value paper, we found that the majority of articles with P values had statistically significant results at .05. This suggests that the .05 P value threshold may have lost its discriminating ability for separating false from true hypotheses.
Closing Thoughts
Please share some closing thoughts on the problem of transparency and reproducibility in scientific research.
Despite all these negative trends, it is important to note that there have been improvements over the past few years in certain areas related to reproducibility and transparency. In a recent study,3 we found that a greater number of papers have conflict of interest and/or funding disclosures. Transparency in this area is critical because funding sources and potential conflicts can influence the way that studies are designed, conducted, and analyzed. In recent years, more studies are also discussing or publicly sharing some parts of their data, which enables future studies to use the data to explore new hypotheses, synthesize evidence, or replicate the findings. These trends represent important improvements related to research transparency. However, detailed protocols, which outline the methods of a study, are rarely available, and the majority of articles continue to claim that they present novel findings.
“The .05 P value threshold may have lost its discriminating ability for separating false from true hypotheses.”— Joshua Wallach, PhD, MS
Tweet this quote
It is promising that support for open science practices has increased over the years and that more journals have reporting guidelines and data-sharing policies. Moving forward, additional efforts will be necessary to continue these trends.
Recently, there has also been discussion about lowering the P value threshold from 0.05 to .005 or abandoning statistical significance. Although statistical testing is problematic, I agree that lowering the threshold as well as improved transparency are helpful measures. ■
DISCLOSURE: Dr. Wallach has received research support through the Meta Research Innovation Center at Stanford (METRICS) and the Collaboration for Research Integrity and Transparency from the Laura and John Arnold Foundation.
REFERENCES
1. Chavalarias D, Wallach JD, Li AH, et al: Evolution of reporting P values in the biomedical literature, 1990-2015. JAMA 315:1141-1148, 2016.
2. Ioannidis JP: Why most published research findings are false. PLoS Med 2:e124, 2005.
3. Wallach JD, Boyack KW, Ioannidis JPA: Reproducible research practices, transparency, and open access data in the biomedical literature, 2015-2017. PLoS Biol 16:e2006930, 2018.