“If we have data, let’s look at it. If all we have are opinions,
let’s go with mine.”
—James Barksdale
In this issue of The ASCO Post, Daniel Vorobiof, MD, and Irad Deutsch, principles at Belong.Life, a patient-oriented website whose self-described mission is to improve patient quality of life and quality of care worldwide through technology, services, data, and artificial intelligence, discuss analyzing patient-related data. Of its platforms, Belong Cancer is the one most relevant to readers of The ASCO Post.
Belong Cancer claims to be “the world’s largest social and professional network for managing and navigating the treatment journey.” It offers diverse services, including interactions with cancer experts, a supportive/interactive patient community, care planning, therapy management, a digital medical and knowledge center binder, personalized content, updates and news flashes, and a clinical trials matching service.
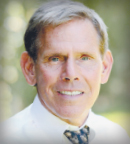
Robert Peter Gale, MD, PhD, DSc (hc), FACP, FRCPI (hon), FRSM
Value of Patient-Centric Data
The authors make several points, the most relevant of which for us relates to patients’ experiences following a cancer diagnosis. They argue that although the medical oncology community, pharma companies, and academic centers are excellent at capturing and analyzing outcomes data (ie, therapeutic response, adverse events, and occasionally quality of life using structured instruments), we are less effective at capturing data on peoples’ personal experiences. As they put it, our main focus is on the cancer rather than the patient. True enough. Consequently, they claim we are missing considerable important data that could improve cancer care.
Another issue highlighted by the authors, one that is well recognized, is outcomes of persons on clinical trials are rarely representative of the universe of people with the cancer being studied. Simply put, what happens after a cancer therapy is approved based on data from clinical trials when applied to so-called real people, not experimental subjects? These revised outcomes are increasingly referred to as real-world evidence.
Lastly, the authors described using artificial intelligence and machine learning to gain insights into patients’ experiences with cancer therapy from large data sets such as Belong Cancer, which claims more than 1 million participants.
Distinguishing Real-World Evidence From Real-World Data
There’s a lot to comment on in this article, but let me tackle a few issues. Almost everyone in the oncology community is familiar with discordances between outcomes of subjects treated on clinical trials and those treated in so-called real-world settings.
For example, in a recent article in Cancer, Phillips et al analyzed discordances for 29 indications for 20 systemic cancer therapies.1 Median survival difference between clinical trials data and real-world data was 5 months (interquartile range = 3–12 months). The hazard ratio for death for someone receiving the same therapy for the same indication—but not in the context of a clinical trial—was 1.58 (95% confidence interval = 1.39–1.80). Simply put, if you receive the same therapy in the real world, you have a 40% to 80% greater risk of dying compared with someone receiving the same therapy on a clinical trial. Wow. And there are lots of other examples of this discordance. There are several reasons for this discrepancy, such as subject selection bias, use of surrogate endpoints for benefit, and better compliance of persons on clinical trials.2
When considering this issue, it’s important to distinguish real-world evidence from real-world data, a topic my colleagues and I discuss elsewhere.3 Data are one thing; deriving reliable evidence from them is quite another and hinges on several factors, such as data quality and how these data are processed into evidence. And this is where artificial intelligence and machine learning enter the picture. This issue has become increasingly important recently, given the U.S. Food and Drug Administration’s approval of some cancer drugs based on real-world data in lieu of results of randomized clinical trials.4
A simple definition of artificial intelligence is every aspect of learning or other feature of intelligence that can in principle be so precisely described that a machine can be made to simulate it. The issue here, of course, is how precisely described is human intelligence. One need only read Thinking, Fast and Slow by Daniel Kahneman to realize other factors impact a precise description of human intelligence.5 Machine learning is a subset of artificial intelligence in which a computer learns from examples rather than being given rules (programming). Unlike humans, who learn to make general and complex associations from few data, machine learning requires much more data to learn the same task. And this is where big data sets like those potentially available in Belong Cancer are useful. (I must point out that machine learning also lacks the uncommon feature of common sense.)
‘The Devil Is in the Details’
So, what should we take away from the perspective by Dr. Vorobiof and Mr. Deutsch? In principle, theirs is a worthy suggestion. However, the devil is in the details. Yes, they have so-called big data, the kind of data needed for machine learning to operate. But how representative are their data of the universe of people with cancer? Are people participating in Belong Cancer like most others with cancer? This seems unlikely. And, if representative, are their inputs reliable, and how can we know? Unlike a clinical trial, auditing is impossible, given participants’ anonymity. Belong Cancer is free to patients with cancer, but it is a for-profit entity. Can we exclude external influences such as pharma? On the technical side, we need to know whether the machine learning processes they propose are to be supervised or not and whether the authors propose advancing to deep learning, a subset of machine learning that uses multiple layers to progressively extract higher-level features from the raw data input.
Overall, I endorse the authors’ proposal. We can provide better cancer care with more patient-oriented data, but we must also acknowledge that in some instances, no data is better than bad data. I look forward to reading the results of the authors’ data-mining of Belong Cancer and similar data sets. Stay tuned.
Dr. Gale is Visiting Professor of Haematology, Centre for Haematology, Imperial College London and Sun Yat-sen University Cancer Center, Guangzhou, China.
Acknowledgment: Dr. Gale acknowledges support from the UK National Institute of Health Research Biomedical Research Centre funding scheme.
DISCLOSURE: Dr. Gale has served as a consultant to NexImmune and Adnexa Pharma, Ascentage Pharma Group, and Antengene Biotech LLC; is Medical Director of FFF Enterprises Inc; is a partner to AZCA Inc; is on the Board of Directors of the Russian Foundation for Cancer Research Support; and is on the scientific advisory board of StemRad Ltd.
REFERENCES
1. Phillips CM, Parmar A, Guo H, et al: Assessing the efficacy-effectiveness gap for cancer therapies: A comparison of overall survival and toxicity between clinical trial and population-based, real-world data for contemporary parenteral cancer therapeutics. Cancer 126:1717-1726, 2020.
2. Heneghan C, Goldacre B, Mahtani KR: Why clinical trial outcomes fail to translate into benefits for patients. Trials 18:122, 2017.
3. Passamonti F, Corrao G, Castellani G, et al: The future of research in hematology: Integration of conventional studies with real-world data and artificial intelligence. Blood Rev 54:100914, 2022.
4. Purpura CA, Garry EM, Honig N, et al: The role of real-world evidence in FDA-approved new drug and biologics license applications. Clin Pharmacol Ther 111:135-144, 2022.
5. Kahneman D: Thinking, Fast and Slow. New York, Farrar, Straus and Giroux, 2013.