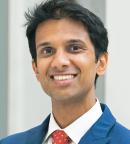
We have to be honest with our patients about what a realistic life expectancy time frame might be, especially when it is extremely different from patients’ expectations.— Ravi B. Parikh, MD, MPP
Tweet this quote
Despite research showing that among patients with cancer, early advance care planning conversations lead to care that is in alliance with patients’ goals and wishes, especially at the end of life,1 most patients die without having discussions about their treatment goals and end-of-life preferences and without the support of hospice care.2 Some common reasons often cited for why these conversations are not taking place include patients’ reticence about discussing end-of-life care and oncologists’ concern that initiating these discussions may cause anxiety and undermine patients’ hope. Another key reason may be that clinicians simply cannot accurately identify patients who would benefit most from these conversations, including those at risk of short-term mortality.
According to Ravi B. Parikh, MD, MPP, Instructor in Medical Ethics and Health Policy at the University of Pennsylvania, prognostic uncertainty and optimism bias may lead patients and oncologists to overestimate life expectancy, delaying important end-of-life discussions. However, the results of a new study by Dr. Parikh and his colleagues evaluating artificial intelligence and machine-learning algorithms to predict mortality among patients with cancer may provide oncologists with a more precise prognostic tool to assess short-term mortality in their patients and to serve as a point-of-care prompt for initiating end-of-life preference conversations.
Dr. Parikh and his colleagues designed the study using data from the electronic health records of 26,525 adult patients who had outpatient oncology care or hematology/oncology care at the University of Pennsylvania Health System and at 10 affiliated community practices. The study found that the machine-learning algorithm accurately identified patients at high risk of dying within 6 months, 51.3% compared with 3.1% for those patients deemed to be at low risk. In addition, in a survey of 15 oncology clinicians at community practices, 100 of 171 patients who had been flagged as being at high risk for short-term mortality by the algorithm were deemed appropriate for a conversation about treatment and end-of-life preferences in the upcoming week.3
The ASCO Post talked with Dr. Parikh about his study results and how they may facilitate timely discussions with terminally ill patients regarding their treatment goals, values, and end-of-life preferences.
Preventing Optimism Bias
Please talk about how prognostic uncertainty and optimism bias may lead patients and oncologists to overestimate life expectancy, thereby putting off end-of-life discussions.
When we shy away from giving patients with advanced cancer a survival prognosis or do not ask patients directly about what they want to know about their survival prognosis, we run the risk of patient-oncologist prognostic discordance. This happens when patients’ survival expectations are different from those of their oncologists. When that happens, patients may want to pursue aggressive therapy in situations in which palliative or hospice care would be more consistent with their goals.
Optimism bias may also be a problem for oncologists, who may overestimate patients’ life expectancy and, thus, delay necessary conversations. This is understandable. Even when you have the cold, hard facts about the course a patient’s cancer is likely to take, and that patient is sitting in front of you, it’s difficult not to lean on the edge of hope. It’s useful to communicate that hope to patients because that can be therapeutic for both patients and doctors. However, we have to be honest with our patients about what a realistic life expectancy time frame might be, especially when it is extremely different from patients’ expectations.
It is a delicate balancing act when you are trying to convey this information all in one visit. As for me, although I tend to be more data driven when giving patients prognostic information, I find I still often overestimate patients’ prognosis.
Even with good intentions, the danger of inaccurately predicting when a patient may die, or not offering prognostic information at all, is that the patient may forgo preparing an advanced-care directive and opt for aggressive treatment that is more harmful than beneficial.
Yes, that is exactly right. The critical shortfall in not delivering a survival prognosis in the advanced-care setting is that we may be depriving patients of the opportunity to participate in serious illness conversations that they otherwise likely would have had if they had a more realistic sense of their life expectancy.
Translating Research Into Clinical Practice
The results from your study suggest that using machine-learning algorithms may be an important first step in identifying patients who could benefit from crucial end-of-life conversations. What surprised you most about your findings?
Guest Editor
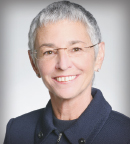
Jamie H. Von Roenn, MD, FASCO
Addressing the evolving needs of cancer survivors at various stages of their illness and care, Palliative Care in Oncology is guest edited by Jamie H. Von Roenn, MD, FASCO. Dr. Von Roenn is ASCO’s Vice President of Education, Science, and Professional Development.
Many of us suspected that machine-learning algorithms would be able to accurately identify a cohort of patients at high risk of short-term mortality. What was most novel and surprising about our study results is finding that the machine’s predictions may actually influence a clinician’s decision to have a timelier conversation with his or her patient about the patient’s treatment goals and end-of-life wishes.
Pessimistically, we thought that many oncologists would either find reasons why the algorithm could be wrong or why, even if the analysis was right in its risk assessment, the patient might not be appropriate for such a conversation. So, we were surprised to find that, of the high proportion of algorithm-identified patients at high risk of death within 6 months, 59% were deemed appropriate by their oncologist to have a serious illness conversation in the upcoming week. That was a promising finding from a proof-of-concept perspective in thinking about deploying this algorithm alongside other types of patient information, such as symptoms and treatment side effects, which are not necessarily associated with mortality and using that information to flag patients for conversations about goals, values, and end-of-life preferences.
Improving the Accuracy of Life-Expectancy Estimates
What is the advantage of using a machine-learning algorithm to predict short-term mortality compared with current methods to predict life expectancy? And what are those current predictive factors?
Currently, there are few accurate methods to estimate life expectancy that are routinely used among patients with terminal cancer. We rely on a number of factors to estimate how long a patient has to live, including population-level survival data for specific cancers and intuition based on our clinical experience. Clinician intuition is a powerful prognostic predictor, but, during a very busy clinic day, it can be difficult to identify which patients would be appropriate for an end-of-life discussion.
A machine-learning algorithm to flag patients in the real-time setting can be a vital decision-support tool for oncologists. Machine-learning algorithms can quickly process a lot of disparate patient information, including current laboratory and imaging test results and recent disease progression, and provide an assessment of the likelihood of a specific patient’s short-term mortality. However, the technology must be used through the eyes of the clinician to determine whether these predictions are 1) useful in practice and/or 2) systematically biased or inaccurate.
Our next step is to launch a randomized clinical study to evaluate machine-learning predictive analytics in real-time, clinical decision-making, for example, to determine whether to begin end-of-life counseling. We have revised the algorithm and how it identifies terminal patients who might be appropriate for a goals and values conversation to evaluate whether having this information increases the number of these conversations in routine clinical practice.
Using this technology may not be able to predict with 100% accuracy when a specific patient may die, but it can identify those at high risk for short-term mortality. It can also ensure that patients and their oncologists have timely conversations regarding the patients’ goals and values, hopefully preventing unwanted aggressive care at the end of life.
DISCLOSURE: Dr. Parikh owns stock or other ownership interests in GNS Healthcare, Google, and Merck; has served as a consultant or advisor to GNS Healthcare; and has been reimbursed for travel, accommodations, or other expenses by the Conquer Cancer Foundation and Flatiron Health.
REFERENCES
1. Brinkman-Stoppelenburg A, Rietjens JAC, van der Heide A: The effects of advance care planning on end-of-life care: A systematic review. Palliat Med 28:1000-1025, 2014.
2. Bernacki R, Obermeyer Z: A need for more, better, and earlier conversations with cancer patients about goals of care. Am J Manag Care 21:166, 2015.
3. Parikh RB, Manz C, Chivers C, et al: Machine learning approaches to predict 6-month mortality among patients with cancer. JAMA Netw Open 2:e1915997, 2019.