A study published in Nature Medicine found that an artificial intelligence program could distinguish between the histologic diagnosis of adenocarcinoma and squamous cell carcinoma.1 Experienced pathologists often struggle to differentiate these tumor types without confirmatory tests. The artificial intelligence tool was also able to determine with a high degree of precision whether several genes linked to lung cancer were present in cells.
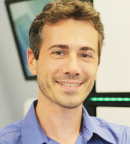
Aristotelis Tsirigos, PhD
The ASCO Post spoke recently with the study’s lead author, Aristotelis Tsirigos, PhD, Associate Professor in the Department of Pathology at New York University (NYU) Langone’s Perlmutter Cancer Center, New York. During his PhD work, Dr. Tsirigos became interested in biology, and he has been doing computational research in medicine for the past 15 years. His laboratory is currently working with biologists to uncover new biologic mechanisms as well as with physicians to develop novel diagnostics and ways to make more accurate treatment decisions.
Artificial Intelligence Terminology
Please explain the differences between machine learning and neural networks.
It’s better to have a broader understanding, beginning with artificial intelligence, which actually includes both disciplines. Artificial intelligence is any action that a computer can do in order to make decisions. An example of artificial intelligence, but not necessarily machine learning, is a rule-based artificial intelligence system. Humans program the artificial intelligence deterministically, using rules that in turn make decisions. For instance, a rule-based system is the way planes fly, having a system of protocols that are followed in a linear fashion. Instead, machine learning is learning by example. It is similar to the way human children learn from their parents, by being shown repeated examples.
There are many different methods within machine learning; one is a neural network, which is a subset of machine learning. They have many layers of computational algorithms. Neural networks have caught our imagination because of their ability to sift through big data repeatedly and make models that are continuously refining themselves.
Study Methods and Key Findings
Please describe your study and its results.
As we reported in Nature Medicine,1our study focused on non–small cell lung cancer (NSCLC) to determine to what extent we could replicate the routine work of pathologists, from biopsy to examining the tissue on a slide under a microscope and making basic diagnostic decisions. It’s important to note that pathologists do a variety of analyses, but we focused on a basic diagnostic decision: determination of the type of lung cancer. We used publicly available data from The Cancer Genome Atlas (TCGA), which has generated comprehensive, multidimensional maps of the key genomic changes in 33 types of cancer.
The TCGA is mainly known for its genomics data, but we realized there were also valuable imaging data. So, with my computational background, I saw this as a great opportunity to test new methods in neural networks to analyze these data. We found about 1,600 usable images of different sizes, some as big as 100,000 pixels in each dimension. We split the images into different classes, using normal lung tissue as a control. We looked at two types of NSCLC: adenocarcinoma and squamous cell carcinoma.
We followed a standard machine-learning procedure to train the neural networks, showing the images to the network and identifying normal tissue as well as the two types of lung cancers. After the initial training process, the models kept improving until we were confident that we had reached maximum improvement. Then we tested the same model on the neural networks using unseen data. We have to ensure that what the model learned is not the result of an artifact or “overfitting.”
We took a two-pronged -approach. We left some of the data out for testing against the machine-learning model. And, being at a medical center, we were able to collect about 300 of our own lung cancer images from biopsies and surgical resections to test against the same model. The study question was: Did the neural network learn something from this highly curated consortium data that could be useful in clinical practice in a real-world scenario?
We were pleased to find that our models performed quite well on the NYU cohort. That was the first step, and then we compared our results with those of pathologists. On the tissue slides, we showed that our neural network model did slightly better than individual pathologists and as good as consensus-arrived results.
Improving Accuracy
How do you improve the accuracy of your machine-learning model?
The neural network model will continue to improve its accuracy as long as it is fed more data. The amount of data is vital, but to avoid the problem of overfitting, a variety of data is needed. If part of an image is out of focus when it is fed to the neural network, it does not understand what out of focus is, so it assumes it is correct. The solution is either to have some sort of quality assessment that rejects out-of-focus images or to teach the network what out of focus looks like. Another issue that could confuse the network may arise from the varying amount of stains doctors use to make histologic images more visible. So, having a variety of data from different data sets helps to expose the neural network to multiple scenarios; then it can problem-solve in real time.
Pathologists Have the Last Word
How do you cull ‘bad’ data from ‘good’ data before you feed it to the neural network?
To do that, we need to work directly with pathologists. All artificial intelligence initiatives involve human experts in any given field. So, expert pathologists are needed to annotate the different slides in terms of specific components or simply to separate them into good or bad samples. It is a collective process that uses data sets and human expertise to feed neural networks with the best possible data. And even though we are making great progress in artificial intelligence diagnostics, the pathologists will have the last word for many years to come. That said, the present level of neural network accuracy will make pathology more efficient and accurate and, perhaps most important, help avoid human errors.
Closing Thoughts
Please share any closing thoughts on this technology.
First of all, I am thrilled that so many doctors within the NYU community are not scared of artificial intelligence. In fact, they often embrace its opportunity and come to us with more data. It is a super important collaboration because doctors actually know the clinical issues and problems. Because of this sharing environment, we have launched other initiatives, not only in cancer but in other diseases.
Our study provides strong evidence that an artificial intelligence approach will be able to instantly determine cancer subtypes and mutational profiles to get patients started on targeted therapies sooner. The end goal is to connect data with the clinic and create better outcomes for patients. It’s exciting work that will truly benefit our health-care system. ■
DISCLOSURE: Dr. Tsirigos is a scientific advisor to Intelligencia AI.
REFERENCE
1. Coudray N, et al: Classification and mutation prediction from non-small cell lung cancer histopathology images using deep learning. Nat Med 24:1559-1567, 2018.