Multiple myeloma is the second most common blood cancer, after non-Hodgkin lymphoma, diagnosed in the United States. In 2020, it is estimated that about 32,270 people will be diagnosed with the cancer, and despite advances in more effective treatments, nearly 13,000 will die of the disease.1 Although significant progress has been made over the past 20 years in understanding the molecular heterogeneity of multiple myeloma, current classifications systems based on fluorescence in situ hybridization (FISH) or karyotyping do not fully capture the diversity observed in the patient population. Response to treatment still varies significantly within the same cytogenetic groups of patients.
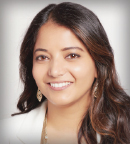
“The Multiple Myeloma–Patient Similarity Network…reveals greater molecular and clinical heterogeneity than current cytogenetic classifications.”— Deepu Madduri, MD
Tweet this quote
Deepu Madduri, MD, of the Department of Hematology and Medical Oncology, Tisch Cancer Institute at Mount Sinai School of Medicine, New York, and colleagues conducted an analysis of multiomics data from the Patient Similarity Network of newly diagnosed patients with multiple myeloma. They identified 3 main patient groups, 12 prognostic subgroups, and potential vulnerabilities in each subgroup based on 5 data types generated from genomic and transcriptomic patient profiling. The Multiple Myeloma–Patient Similarity Network (MM-PSN) classification uncovered novel associations between distinct hallmarks of the cancer with significant prognostic implications and allowed further refinement of risk stratification.2 The study findings were presented virtually during the 2020 American Society of Hematology (ASH) Annual Meeting & Exposition.
Study Methodology
The researchers used whole-genome sequencing, whole-exome sequencing, and RNA sequencing to generate data from bone marrow aspirates (CD138-positive cells) and peripheral blood (control) of 655 newly diagnosed patients with multiple myeloma enrolled in the MMRF CoMMpass Study. Copy number alterations and translocations were identified from whole-genome sequencing data, somatic single nucleotide variations were identified from whole-exome sequencing data, and gene-level counts and gene fusions were inferred from RN sequencing data.
In the MM-PSN, the researchers integrated five data types: gene expression, gene fusions, copy number alterations, somatic mutations, and chromosomal translocations with clinical data from each patient. Spectral clustering of MM-PSN data revealed 3 main patient groups and 12 subgroups of similar patients, each characterized by specific patterns of genetic and molecular features. Group 1 included 357 patients (54.5%), mainly enriched for hyperdiploidy, MYC translocations, and NRAS mutations, and comprised 4 subgroups. Group 2 included 166 patients (25.3%), which were overall enriched for MMSET and MAF translocations. Group 3 included 132 patients (20.15%) and comprised 3 subgroups.
Key Results
Survival analysis by the researchers revealed several novel prognostic findings that improve on current cytogenetic risk classification systems. For example, although patients with hyperdiploidy are considered to have a better prognosis, their analysis found that concurrent gain(1q) identifies a subgroup of these patients at higher risk (1c) for relapse. Similarly, the MMSET translocation t(4;14) is currently considered to confer a poor prognosis and identifies high-risk in the Revised Multiple Myeloma International Stage System. The researchers’ findings demonstrate significant heterogeneity within this group, in which patients in subgroup 2e [with MMSET translocation +gain(1q)] had the poorest prognosis in terms of both progression-free and overall survival in the entire cohort, whereas those in subgroup 2a (with MMSET translocation alone) had a significantly better prognosis, comparable to that of the hyperdiploidy subgroups.
This finding has important implications, according to the researchers, because it shows that MMSET translocation does not always confer a poor prognosis and that only a fraction of patients with concurrent gain(1q) are at high risk. Additionally, their model revealed a protective effect conferred by gain(15q), whose presence determined significantly longer progression-free and overall survival, even after adjusting for gain(1q).
KEY POINTS
- The Multiple Myeloma–Patient Similarity Network (MM-PSN) classification uncovered novel associations between distinct hallmarks of the cancer with significant prognostic implications and allowed further refinement of risk stratification.
- The MM-PSN integrates multiomics data from patients with multiple myeloma and reveals greater molecular and clinical heterogeneity than current cytogenetic classifications.
- Ongoing research is focused on deeper investigation of the molecular mechanisms driving each subgroup of patients with myeloma and further validation of treatment options identified for specific subgroups of patients.
Further analysis of pathways enriched by gene expression in the subgroups found specific enrichment for interleukin signaling in group 1; growth, proliferation, and differentiation pathways in group 2; and inflammation and other immune-related pathways in group 3. The researchers further identified therapeutic vulnerabilities and treatment options that may be important in a subgroup-specific manner—for example, CDK6 in the MAF translocation subgroup 2b, IGF1R in the MMSET translocation +gain(1q) subgroup 2e, and CCND2 across several subgroups of the hyperdiploidy and MMSET/MAF translocation groups. Drug repurposing analysis identified novel potential therapeutic options in a subgroup-specific manner, such as eportin 1 (XPO1) inhibitors in the hyperdiploidy subgroup 1b and MAPK inhibitors in the MMSET translocation +gain(1q) subgroup 2e.
Clinical Significance
“In conclusion, MM-PSN integrates multiomics data from patients with multiple myeloma and reveals greater molecular and clinical heterogeneity than current cytogenetic classifications. Ongoing research is focused on a deeper investigation of the molecular mechanisms driving each subgroup and further validation of treatment options identified for specific subgroups,” concluded the study authors.
DISCLOSURE: Dr. Madduri has served as a consultant to Foundation Medicine, Kinevant, GSK, Sanofi, Legend, Takeda, Bristol Myers Squibb, and Janssen. For disclosures for the other study authors, visit ash.confex.com.
REFERENCES
1. American Cancer Society: Key Statistics About Multiple Myeloma. Available at www.cancer.org/cancer/multiple-myeloma/about/key-statistics.html. Accessed December 7, 2020.
2. Bhalla S, Melnekoff D, Keats JJ, et al: Patient similarity network of multiple myeloma identifies patient subgroups with distinct genetic and clinical features. 2020 ASH Annual Meeting & Exposition. Abstract 3154. Presented December 7, 2020.