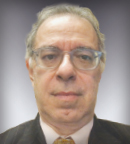
David F. Yankelevitz, MD
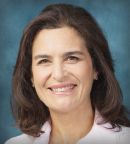
Ella Kazerooni, MD, MS
“Artificial intelligence [AI] will be used in all aspects of [lung cancer] screening…, and it continues to get better,” commented topic overview speaker David F. Yankelevitz, MD, Professor of Radiology at the Icahn School of Medicine at Mount Sinai, New York, at the Quantitative Imaging Workshop XXI: Advancing Routine Clinical Use of Health Information From Thoracic CT Screening for Public Health.1
Together with Ella Kazerooni, MD, MS, Professor of Radiology and Internal Medicine at the University of Michigan Medical School, Ann Arbor; Artit Jirapatnakul, PhD, Associate Professor of Diagnostic, Molecular, and Interventional Radiology at Icahn School of Medicine at Mount Sinai; and Mario Silva, MD, PhD, Assistant Professor of Radiology at the University of Parma, Italy, and Adjunct Assistant Professor of Radiology at the University of Massachusetts Chan Medical School, Worcester, the expert panel discussed the deployment of such technology and its potential impact on the field.
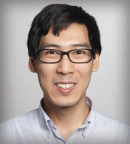
Artit Jirapatnakul, PhD
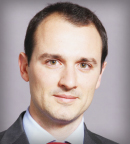
Mario Silva, MD, PhD
The Need for Data
“[Regarding nodule detection, growth assessment, and characterization], what is missing is not so much the AI development as it is the data,” Dr. Yankelevitz commented. He noted that most of the algorithms for lung cancer have been trained on the “old” Lung Image Database Consortium (LIDC) and National Lung Screening Trial (NLST) data sets.
To further emphasize his point, Dr. Yankelevitz discussed the results of an unpublished analysis of NLST data conducted by Dr. Jirapatnakul, which showed that 85% to 90% of computed tomography (CT) scans used a slice thickness of over 1.25 mm. “That doesn’t meet anybody’s standard anymore for what we should be doing for lung cancer screening, and yet virtually every product out there is built on this,” he remarked.
A separate analysis excluded both CT scanners using an edge-enhancing kernel and those with known spatial warping, which respectively comprised 40% and 22% of the initial sample, according to Dr. Yankelevitz.2 He thus stated: “You clearly cannot rely on [that data to develop] modern AI that is going to change the world, so our focus needs to be on getting massive databases where we can train the AI.”
Focus on Measuring Growth
Artificial intelligence “just continues to get better” at finding nodules, according to Dr. Yankelevitz. The question thus becomes: “How much better does it have to get?”
“I think there is still a lot of room for improvement [in evaluating] change over time and how this is looked at,” Dr. Yankelevitz commented. “The ability to measure growth and change is absolutely fundamental to what cancer is and how we should define things as being cancer.”
He emphasized the complexity of volumetrics, with variability in measurements observed across scans even in the best-case scenarios. Interactions among multiple factors, including the nodule size, complexity, location, scanner, scan parameters, and measuring software, may influence such uncertainties in growth assessment.
Furthermore, regarding the optimal time interval for obtaining a follow-up diagnostic scan, Dr. Yankelevitz stated that “a balance between accuracy of growth measurement and decrease in prognosis is needed.” Biomarker testing may enable earlier diagnoses compared with waiting for a growth assessment scan, he added, acknowledging that this benefit must be balanced against the rates of potential measurement errors.
Concluding this topic of discussion, Dr. Yankelevitz emphasized the importance of CT image quality. “[Modern scanners] will give you better assessments of growth,” he remarked.
Dr. Yankelevitz stated that the “dream” is the complete automation of lung cancer screening. The U.S. Food and Drug Administration approvals of AI-powered chest health and bone mineral density evaluations have demonstrated the potential for similar advancements in this field.
“The whole idea of lung screening being a health check is where I think this is going to head in the next few years,” he concluded.
Low-Dose CT Lung Screening: AI Opportunities
Each of the panelists was asked the following question: “If you had the ability to apply hundreds of billions of dollars [to AI in the context of lung cancer screening, where] would you put the emphasis to have an impact in the next 3 to 5 years?” The areas were ranked on a numerical scale, with 1 being the lowest priority and 10 being the highest.
Dr. Kazerooni emphasized risk assessment, giving it a score of 9: “We are pretty good with non–imaging-based risk assessment, and the addition of imaging-based AI risk assessment will really get us there,” she said. The remaining areas, ranked in descending order, were detection (score = 8), patient communication (8), therapy (6), and differential diagnosis (4).
According to Dr. Jirapatnakul, “reducing the burden on the radiologist is key.” He thus ranked detection and patient communication highly, with scores of 10 and 8, respectively. Lower scores were assigned to risk assessment (7), therapy (7), and differential diagnosis (5).
Dr. Silva primarily opted to allocate his money to risk assessment (9), citing a need for better tools, with therapy (8) and patient communication (8) as his next priorities. He added that he would put “a bit more money into [differential diagnosis (7)] than detection [(6)],” because “improvement of volume doubling time measurement is what we need…, [and] lung cancer detection is already achieved.”
The average panelist scores for risk assessment, detection, differential diagnosis, therapy, and patient communication were 8.3, 8.0, 5.3, 7.0, and 8.0, respectively. However, as previously stated by Dr. Yankelevitz, there is a need for more data.
DISCLOSURE: Dr. Yankelevitz is named inventor on several relevant patents and patent applications; has served on a medical advisory board for Carestream Health, LungLife AI, Median Technology, and HeartLung; has served on an advisory panel for Pfizer; and is a shareholder in Accumetra and HeartLung. Dr. Kazerooni, Dr. Jirapatnakul, and Dr. Silva reported no conflicts of interest.
REFERENCES
1. Yankelevitz DF, Silva M, Jirapatnakul A, et al: How will AI impact risk assessment, small nodule detection, differential diagnosis, and patient communication? Where will we see the biggest impact? Quantitative Imaging Workshop XXI: Advancing Routine Clinical Use of Health Information From Thoracic CT Screening for Public Health. Session 3: Deploying Artificial Intelligence for Lung Cancer Screening. Presented November 14, 2024.
2. Henschke CI, Yankelevitz DF, Yip R, et al: Tumor volume measurement error using computed tomography imaging in a phase II clinical trial in lung cancer. J Med Imag 3:035505, 2016.