The more senior of this duo grew up with prognostication by disease stage and was taught that all stage IV cancers behaved the same. In the past 3 decades, we have become much more cognizant of the heterogeneity in outcome within stage. Individual Kaplan-Meier plots by stage separate well but hide the fact that there are many early deaths in early stages of cancer and a number of long-term survivors among patients with advanced stages of the disease.
We noted this point when we developed a nomogram for the outcome of resected gastric cancer1 and compared the nomogram-predicted survival with survival predicted by the American Joint Committee on Cancer (AJCC) staging system. Although stage I and stage IV were associated with comparable AJCC stage and nomogram survival, within intermediate stages IB through IIIB, individual nomograms predicting survival could vary by 60% to 80%.
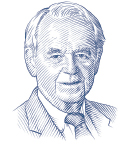
Murray F. Brennan, MD
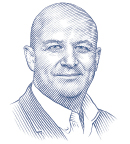
Mithat Gönen, PhD
This variability was also recognized within stage IV resected colorectal metastases,2 where within-stage prognostic 5-year tumor recurrence predictions varied from 14% to 60%, depending on the combination of adverse risk factors that make up the component index score. This is equally true when we look at survival following the recurrence of a resected extremity sarcoma3: a < 5 cm, low-grade recurrence occurring after 16 months has a 4-year disease-specific survival of 81%, whereas a > 5-cm local recurrence that is high-grade and occurs within 16 months has a disease-specific survival rate of 18%. Intuitively, that makes sense. Aggressive recurrence would be expected to define aggressive biology and the converse. But in classifications of stage and prognostic clinical scores, the individual variables that create the prediction are all weighted equally. Nomograms help by weighting variables but do not address the consequences of combinations of variables.
The less senior of us grew up with prediction by regression models. These tried-and-true models on which nomograms are based are very good at capturing the “low-hanging fruit,” like the prognostic effects of size, the number of positive nodes, and site-specific histologic criteria. The focus in these models is on main (or marginal or direct) effects of variables, not the effects of combinations. This helps with simplicity and interpretability but might miss subtle prognostic effects. They provide us with a reliable silhouette of the future, but details remain blurry and hence the heterogeneity in our predictions.
Managing the Discrepancies
So, how to deal with this heterogeneity in outcome within classical stage or prognostic indices? In nomograms, we try to provide a solution with the confidence limits of the individual prediction. Those limits will be defined by the number of observations that are contained for the specific individual. An attempt to address this occurs when we go from a sarcoma-specific nomogram to a histology-specific nomogram.4 Unfortunately, as we progressively define each tumor type based on a unique signature, we have ever-smaller data sets with ever more imprecision.
One option is to embrace more complex artificial intelligence solutions—the Reverend Bayes would be pleased. He just wanted to allow experience to guide the question of validity. What we are searching for is how to encompass the significance of interrelated variables. That is to say, not only is the solitary variable significant, but some combinations of variables have an equal or perhaps greater impact than the component variable. A statistician would model this with an interaction between variables in a regression model, but the number of parameters in these models grows exponentially, and things get quickly out of hand.
“[Regression models] provide us with a reliable silhouette of the future, but details remain blurry and hence the heterogeneity in our predictions.”— Murray F. Brennan, MD, and Mithat Gönen, PhD
Tweet this quote
Another possibility is the Optimal Classification Tree, as promoted by Bertsimas.5,6 It is not a new concept,7 but it is highly dependent on advances in artificial intelligence and computing power to be realistic. Although this strategy is still binary, leading with the strongest predictor of outcome, a sequential combination of factors can be obtained that accurately predicts disease-specific survival at a particular point in time, much like branches of a tree. The method does not weight components in a linear fashion, but it can provide variables that—given one is present or absent—predict the next most important variable to define the ultimate outcome. That process conveys the combination and hence emphasizes the interdependence of individual variables.
This fundamental idea of building classification trees, also called recursive partitioning, is prone to overfitting and requires careful validation, but with the right data set and a rigorous approach, might turn the silhouette into a portrait. Once we learn how to grow a tree for making predictions, we are in a position to make a forest out of it. Random forests—so-called because they contain many of these classification trees, each simultaneously based on a randomly perturbed version of the original data set—tend to have higher predictive power but less interpretability. With one tree, we can see the branches and leaves clearly. With thousands of them, we literally cannot see the trees for the forest. We have just slid from the world of parsimonious, transparent, but only moderately powerful predictive models to black box algorithms that trade accuracy for interpretability.
Closing Thought
What does a clinician do in the face of heterogeneity that may require complex computational intervention? Inspired by the old Russian proverb, “Trust, but verify” (doveryai, no proveryai), we suggest, “Embrace, but question.” Welcome modern methodology, but keep critical thinking. Seek a balance between reducing and explaining heterogeneity. The road ahead is like a fractal—it looks regular and smooth from above but has many twists and sharp turns.
Dr. Brennan was Chairman of Surgery at Memorial Sloan Kettering Cancer Center (MSK) for 21 years and is now Senior Vice President of International Programs and Director of the Bobst International Center, MSK, New York. Dr. Gönen is Chief of the Biostatistics Service at MSK.
Disclaimer: This commentary represents the views of the author and may not necessarily reflect the views of ASCO or The ASCO Post.
DISCLOSURE: Dr. Brennan and Dr. Gönen reported no conflicts of interest.
REFERENCES
1. Kattan MW, Karpeh MS, Mazumdar M, et al: Postoperative nomogram for disease-specific survival after an R0 resection for gastric carcinoma. J Clin Oncol 21:3647-3650, 2003.
2. Fong Y, Fortner J, Sun RL, et al: Clinical score for predicting recurrence after hepatic resection for metastatic colorectal cancer: Analysis of 1001 consecutive cases. Ann Surg 230:309-321, 1999.
3. Eilber FC, Brennan MF, Riedel E, et al: Prognostic factors for survival with locally recurrent extremity soft tissue sarcomas. Ann Surg Oncol 12:228-236, 2005.
4. Dalal KM, Kattan MW, Antonescu CR, et al: Subtype specific prognostic nomogram for patients with primary liposarcoma of the retroperitoneum, extremity, or trunk. Ann Surg 244:381-391, 2006.
5. Bertsimas D, Dunn J: Optimal classification trees. Mach Learn 106:1039-1082, 2017.
6. Bertsimas D, Dunn J, Velmahos GC, et al: Surgical risk is not linear: Derivation and validation of a novel, user-friendly, and machine-learning-based predictive OpTimal Trees in Emergency Surgery Risk (POTTER) calculator. Ann Surg 268:574-583, 2018.
7. Breiman L, Friedman J, Stone CJ, et al: Classification and regression trees. New York, Taylor & Francis, 1984.