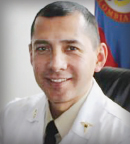
Luis Eduardo Pino Villarreal, MD, MSc, MBA
The 2021 ASCO Annual Meeting was presented totally virtually again due to the persistence of the COVID-19 pandemic. Nevertheless, the meeting held widespread interest, and we were able to attend an event with important changes for the practice of oncology. Compared with 2020, fewer “next-generation oncology” works were presented in 2021, but in my opinion, the quality of these presentations was significantly improved.
This year, I found 86 print abstracts and 5 additional oral presentations related to the following search terms: artificial intelligence (AI), machine learning, deep learning, and deep neural networks. I decided not to use the terms “digital platform” or “applications” because I wanted to focus specifically on cognitive developments in oncology. I will begin with highlights of the oral presentations.
Artificial Intelligence and Related Technologies
There were some remarkably interesting oral presentations on advances in artificial intelligence. The first of them was that of Tommaso Mansi, PhD, of Siemens Healthineers,1 who emphasized that the momentum of these technologies in health care is related to a greater capacity in algorithmization, computing, connectivity, and volume of data. According to Dr. Mansi, these developments should focus on three fundamental goals: (1) personalized therapies for improved outcomes; (2) decreased variability and improved efficiency; and (3) improved access to care.
Optimization in risk and outcome prediction, process optimization, and ex vivo modeling such as the use of digital twins (virtual simulations to aid in personalizing care) are also exciting prospects for the implementation of next-generation oncology. Finally, some big ideas about the creation of value using artificial intelligence were remarkable: increasing area under the curve (AUC) associated with this technology (eg, to manage uncertainty and bias), enabling precise inference of complex data, and generating value conditioned by scale (better data analytics).
Jhanelle Elaine Gray, MD, of Moffitt Cancer Center, chaired the session titled, “Artificial Intelligence: Optimizing Cancer Care Using Imaging and Pathology,” in which the following three abstracts were presented.
Chi Wah Wong, PhD, of City of Hope Comprehensive Cancer Center, and colleagues used a gradient-boosted tree model to classify survival as above or below 180 days with two-loop nested cross-validation in patients with recurrent high-grade glioma treated with chimeric antigen receptor T-cell therapy. The investigators predicted that patients with larger tumor surface area, tumor volume, and age have reduced survival scores, whereas patients with larger IL-13Rα2 and tumor sphericity have increased survival scores.2 Patients were stratified into two distinct prognostic subgroups (30 patients in each group) using the survival scores obtained from the outer loop, with a log-rank test P < .01. For the inner validation loop, hyperparameter tuning was used. For prediction explanations, they adopted the Shapley additive explanations (SHAP) framework, a game theory approach used to explain the output of any machine learning model. This work relies mainly on the use of the SHAP framework to predict analytics in real-world patients.
Michael R. Tomaszewski, PhD, of Moffitt Cancer Center, and colleagues relied on a negative study for soft-tissue sarcomas (SARC021), which evaluated the efficacy of evofosfamide.3 They tested whether an inclusion/exclusion model based on radiomic analysis and relevant clinical covariates could have been employed to result in a significant treatment benefit of the evofosfamide-plus-doxorubicin combination compared with standard doxorubicin monotherapy. A total of 163 radiomics features were extracted from lung metastases in 303 patients from the SARC021 trial, divided into demographically matched training and test sets. A radiomic parameter, short-run emphasis, was identified as the most informative feature. When combined with tumor histology and smoking history information, an enriched subset (42%) of patients had a longer overall survival in evofosfamide/doxorubicin vs doxorubicin-alone groups (P = .01, hazard ratio = 0.57, 95% confidence interval [CI] = 0.36–0.90), overperforming a clinical-only approach. In the future, these findings could potentially change the way clinical trials allocate patients to specific study arms, using radiomics and clinical features based on a predictive model such as this one.
Sanja Dacic, MD, PhD, of the University of Pittsburgh Medical Center, and colleagues, developed an AI-powered digital pathologic response assessment tool in line with manual consensus recommendations to evaluate pathologic response in resection specimens after immunotherapy in patients with non–small cell lung cancer (NSCLC) from the LCMC3 study.4 Machine learning models were trained and validated by the PathAI research platform using digitized hematoxylin and eosin–stained tumor sections. Interreader agreement among one local and three central pathologists for manual pathologic response was good (n = 127; interclass correlation coefficient = 0.87; 95% CI = 0.84–0.90). Agreement was 91% (κ = 0.82) on manual major pathologic response and 98% (κ = 0.88) on pathologic complete response. Six patients had a unanimous pathologic complete response. Digital and manual pathologic responses were strongly correlated (n = 135, Pearson r = 0.78), and digital pathologic response demonstrated an outstanding predictability for manual major pathologic response (area under the receiver operating characteristic curve = 0.975). This trial might open the door to a hybrid central assessment of pathologic outcomes in large studies of immuno-oncology or other technologies.
At the end of this session, there was an interesting discussion about the reliability of these artificial intelligence methods in the real world and the timeline for effective implementation in the clinic. Pathologists and radiologists are the physicians who currently might adopt AI tools in their daily work most rapidly.
Personalizing Therapies for Improved Outcomes
I would like to classify the abstracts I reviewed according to the concept previously described by Dr. Mansi regarding the three goals of artificial intelligence in health care. A total of 18 works were focused on the first of those goals—personalizing therapies for improved outcomes. I chose the following abstracts because of their potential for significant contributions to the field:
Daniel Ure, MS, and Gary Altwerger, MD, of Aspira Women’s Health, presented work with the serum-based Multivariate Index Assay 3G (MIA3G) to improve the prediction of adnexal mass risk of malignancy.5 MIA3G was constructed as a deep feedforward neural network (ie, an artificial neural network that moves information forward to output nodes, without recurrent cycles or loops) using seven serum biomarkers. The neural network was trained and validated with a divided cohort and untested samples set aside. MIA3G achieved a sensitivity of 91.3% (CA-125 sensitivity = 65.2%) and higher early-stage detection (83% vs 50% with CA-125). I can’t wait for a large, prospective validation study using this deep feedforward neural network.
Raquel Reisinger, MD candidate, at the University of Utah School of Medicine, and colleagues created a Bayesian network using 17 variables as a genomic predictor of response in metastatic urothelial carcinoma receiving systemic therapy (n = 174).6 The Bayesian network revealed a strong positive interdependence between immuno-oncology response rate and tumor mutation burden ≥ 10 mutations/Mb or a mutated PIK3CA gene. These two variables could be included in the initial evaluation of patients with metastatic urothelial carcinoma who may be candidates for immuno-oncology therapies.
Juntaro Matsuzaki, PhD, of Keio University, Tokyo, and colleagues developed a strategy for machine learning–based detection of multiple cancers with circulating microRNA profiles in blood.7 This project is part of a national project to develop a circulating microRNA database in Japan. Serum samples of patients were collected and processed for microRNA expression profiles using next-generation sequencing, and results were used as a large training set for a machine learning model in a 4:1 ratio. Details of the machine learning model architecture and validation were not provided, but the diagnostic accuracy was 88% for five cancer types (breast, lung, colorectal, stomach, pancreatic). MicroRNA automated analysis has great potential for higher sensitivity, even in the early stages of cancer.
Rebecca Lee, MD, of the Christie NHS Foundation Trust, London, and colleagues created a machine learning tool (COVID-19 Risk in Oncology Evaluation Tool, or CORONET) to determine which patients may be suitable for immediate hospital discharge and ambulatory care and which patients may be more likely to have severe infection.8 CORONET was trained using a recursive feature elimination model based on a SHAP framework (10 features were selected). A random forest regression model was used and then validated using a fourfold cross-validation. Performance of the model was measured as an AUC of 0.82 for hospital admission, 0.85 for oxygen requirement, and 0.79 for death.
The model is available at https://coronet.manchester.ac.uk as a clinical reasoning support tool for prospective use. I plan to start using it.
Decreasing Variability and Improving Efficiency
Alexander S. Rich, PhD, of Flatiron Health, and colleagues developed a unified deep learning model for determining initial and advanced NSCLC diagnoses and their date from electronic medical record text.9 This is a natural language processor project using a set of sentences containing NSCLC-related keywords to train a deep learning model. Sentences were processed using a gated recurrent unit neural network followed by an attentional network that integrated information across sentences. Deep learning model output predicted whether a patient had been diagnosed with NSCLC and the diagnosis date. Final sensitivity was 0.98 and 0.95 for initial and advanced diagnoses, respectively. This is a good achievement for a natural language processor used in an unstructured and overly complex world such as NSCLC diagnosis. I think this model could be improved using advanced techniques such as Transformer-XL, a newer type of architecture that enables natural language understanding beyond a fixed-length context. An intelligent capture of text is still a dream for oncologists, but data harmonization projects such as m-CODE from ASCO will be essential to create better natural language processors.
Improving Access to Care
Alexi A. Wright, MD, MPH, of Dana-Farber Cancer Institute, discussant at another oral session, presented an attractive proposal based on telehealth and machine learning for COVID-19 in patients with cancer.10 It connects reasoning support tools (in this case, the CORONET tool mentioned previously) in a remote patient monitoring system that captures and predicts not only features related to clinical outcomes, but also electronic patient reported outcomes, and provides system alerts and teleconsultation as well. We may soon see this framework in a restructured version of oncology care.
Ajeet Gajra, MD, FACP, of Cardinal Health Specialty Solutions, and colleagues showed us Jvion CORE, a commercially available artificial intelligence tool that generates insights into risk for short-term mortality (within 30 days) among patients with cancer.11 Those insights were communicated to providers to potentially intervene and modify care for patients at medium to high risk based on established practice pathways. Insights related to higher short-term mortality risk included emergency department visits, admissions, intensive care unit care, and radiation or chemotherapy in the past month. The use of this algorithm improved palliative care coverage by providers and decreased early mortality in some subgroups.
Samir Courdy, Senior Vice President of Informatics at City of Hope Comprehensive Cancer Center, and colleagues reported on -POSEIDON, an enterprise-wide platform and precision medicine program to unlock the research potential and clinical value of complex and unique data sets by combining patient data with comprehensive genomic profiling and proprietary analytics.12 This platform enables investigators to access and visualize data from clinical and multiomics data and provides an engine that can be utilized for cohort discovery and exploration and preliminary feasibility testing to deriving patient-specific insights based on real-world data and real-world evidence. Patients provide consent through an institutional review board–approved protocol with active, opt-in participation. Natural language processing tools are deployed to automate and structure valuable data elements from unstructured documents, even clinical notes. This tool includes 175,000 patients and is an exceptional resource for collaborative real-world data and real-world evidence studies.
In Conclusion
I apologize for being unable to mention many other excellent studies in the field due to space constraints. That said, 2021 continues to be an atypical but hopeful year, not only due to new therapies of high impact and value for our patients, but also given the immense horizon of research in next-generation oncology concepts being advanced. See you next year.
DISCLOSURE: Dr. Pino Villarreal has served as an advisor/consultant to AstraZeneca, Roche, MSD, and BMS; and has served as a speaker for AstraZeneca, Roche, and BMS. He is also founder and CMO of MedzAIo and MAIA®.
REFERENCES
2. Wong CW, et al: Explainable prediction of survival using clinical, molecular, and radiomic profiles in recurrent high-grade glioma patients treated with CAR T-cell therapy. 2021 ASCO Annual Meeting. Abstract 104.
3. Tomaszewski MR, et al: Imaging-based patient inclusion model for clinical trial performance optimization. 2021 ASCO Annual Meeting. Abstract 105.
4. Dacic S, et al: Artificial intelligence–powered pathologic response assessment of resection specimens after neoadjuvant atezolizumab in patients with non-small cell lung cancer. 2021 ASCO Annual Meeting. Abstract 106.
5. Ure D, et al: Serum-based assay for adnexal mass risk of ovarian malignancy. 2021 ASCO Annual Meeting. Abstract 5551.
6. Reisinger R, et al: Genomic predictors of response to PD-1 axis inhibitors in metastatic urothelial cancer patients using machine learning analysis of tissue comprehensive genomic profiling. 2021 ASCO Annual Meeting. Abstract 4542.
7. Matsuzaki J, et al: Machine learning-based multiple cancer detections with circulating miRNA profiles in the blood. 2021 ASCO Annual Meeting. Abstract 3037.
8. Lee R, et al: CORONET. 2021 ASCO Annual Meeting. Abstract 1505.
9. Rich AS, et al: Extracting non-small cell lung cancer diagnosis and diagnosis dates from electronic health record text using a deep learning algorithm. 2021 ASCO Annual Meeting. Abstract 1556.
11. Gajra A, et al: Outcomes among patients with cancer identified as being at risk for 30-day mortality using augmented intelligence. 2021 ASCO Annual Meeting. Abstract 12031.
12. Courdy S, et al: The City of Hope POSEIDON enterprise-wide platform for real-world data and evidence in cancer. 2021 ASCO Annual Meeting. Abstract e18813.
Dr. Pino Villarreal is a medical oncologist and hematologist at Fundación Santafé in Bogotá, Colombia. He is also Professor of Medicine at Unversidad de los Andes as well as Chief Medical Officer and Cofounder of a digital health-care company, MedZaio.