Artificial intelligence (AI)—computational analytics with routine imaging via radiology or pathology—can advance precision medicine in breast cancer, specifically by predicting response to therapy and calculating prognosis, according to a pioneer in the field, Anant Madabhushi, PhD, of Emory University School of Medicine, Atlanta.1 Dr. Madabhushi holds many titles, including Robert W. Woodruff Professor in the Departments of Biomedical Engineering, Radiology & Imaging Sciences, Biomedical Informatics, Urology, Radiation Oncology, Pathology, Computer Science & Informatics and Global Health, and Director of the Emory Empathetic AI for Health Institute.
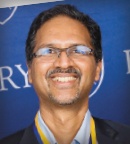
Anant Madabhushi, PhD
In the Scientific Keynote Address at the 2024 Miami Breast Cancer Conference, Dr. Madabhushi described his team’s cutting-edge research using computational analyses aimed at improving cancer outcomes.1
Meeting the Challenges
“One of the biggest challenges is the issue of overdiagnosis and overtreatment of a number of cancers,” Dr. Madabhushi stated. “A case in point is prostate cancer, where 1 in 7 men will be diagnosed with it, but only 1 in 40 will actually die of it. Yet we throwthe proverbial kitchen sink in terms of treatment at a number of these cancers, even though many of them are indolent and less aggressive,” he added.
Novel treatments, such as immunotherapy, have enhanced outcomes but do not work in all patients and often create “financial toxicity,” so more streamlined approaches are needed. “Our group is looking at the role of AI, not just in developing better diagnostic tools but also better prognostic and predictive tools, so we can appropriately tailor therapy,” Dr. Madabhushi said.
“The point is there’s so much information we are not currently capturing, and with the power of AI, we can pull out features in areas we have not been typically used to evaluating,” he commented.
Latest AI Approaches
Newer “advanced hand-crafted engineered AI” approaches go beyond the more traditional “deep learning” algorithms of AI. These powerful programs do not require the abundance of data input that is the hallmark of deep learning; they can learn directly from annotations of the cancer target of interest, can identify patterns that correspond to those targets, and then can find those targets on new, previously unseen images.
Dr. Madabhushi’s group is conducting research based on these programs. They are also particularly interested, he added, in “addressing the reproducibility crisis in AI”—ie, the inability of AI algorithms to reliably be applied in sites beyond where they were developed. “Part of the reason is many of these neural network algorithms are very opaque,” he explained. “The data are provided to the AI algorithm, the AI learns some patterns, but we don’t have a grasp of what these patterns are or what the AI is learning. This issue of reproducibility with AI is really important.”
The solution could be more “explainable AI approaches” focusing on patterns that are “more interpretable and more intuitive” to clinicians and therefore more trustworthy. These approaches should be validated within clinical trials and among institutions, before they can be trained for clinical deployment, Dr. -Madabhushi suggested. Access, affordability, and equity are also important considerations in building AI tools for precision medicine. “If you develop algorithms that are biased and not exposed to a diversity of populations, they will simply not work across all patient populations,” he noted.
To further the goals of accessibility and affordability, much of his team’s work has focused on routinely acquired data, such as computed tomography (CT), magnetic resonance imaging (MRI) scans, and histopathology images. They employ radiomics and pathomics to extract patterns from those images that can be correlated with outcomes. With “digital phenotyping,” the researchers are applying AI to routine biopsy images to identify different tissue compartments (ie, stroma, epithelium, individual nuclei, and lymphocytes) whose architectural arrangement, shape, and interplay may ultimately be associated with treatment response and outcomes. Similar approaches can also be applied to radiologic images.
Prognostic Power of AI
For example, in patients with early-stage, estrogen receptor–positive breast cancer, investigators have found that collagen fibers were more “ordered” in women with shorter disease-free survival, whereas “disorder” was associated with longer survival.2 The features remained prognostic in a regression model, and their performance was validated in a completed clinical trial data set.
Dr. Madabhushi’s group also found that computationally derived image features from H&E (hematoxylin and eosin) stains (related to nuclear morphology, mitotic activity, tubule formation) added prognostic value to Oncotype DX risk categories in estrogen receptor–positive, node-negative breast cancer.3 Using these features, they trained a Cox regression model they labeled an “image-based risk score,” which proved to be significantly prognostic of disease-free survival. In addition, the image-based risk score reliably stratified risk within the high Oncotype DX risk categories, potentially providing more granular risk stratification than offered by the assay alone, he explained.
Other research has shown that spatial features of tumor-infiltrating lymphocytes may identify distinct risk groups and/or predict outcomes within HER2-positive breast cancer, triple-negative breast cancer, and immunotherapy-treated non–small cell lung cancer (NSCLC).
Using Radiology Images
Much of Dr. Madabhushi’s work pertains to radiomics, which is the use of AI algorithms to detect patterns from standard radiologic images. He and his colleagues have shown that by using MRI scans of glioblastomas, they can distinguish radiation necrosis from recurrence (a clinical challenge). They see “more entropy and more morphologic heterogeneity” in tumor recurrences and less entropy in radiation necrosis.
“Here’s an example of taking your standard radiology exam and with the application of AI convert that into something visually intuitive, that can help guide the management of patients with glioblastoma,” he continued. Similarly, his team applied the “entropy” identification approach to metastatic HER2-positive breast cancer and identified radiomics features that not only predicted response to CDK4/6 inhibitors but were associated with overall survival.4
They have also introduced a new class of imaging biomarkers that measure the shape and architecture of the tumor-associated vasculature. “We’ve gotten very interested in looking at regions outside the tumor,” Dr. Madabhushi explained. “We began asking whether there is something with regard to the tumor-associated vasculature that we can extract from a standard MRI using AI. Can we learn something from the morphology and from the shape and appearance of the tumor-associated vasculature?”
In a large breast cancer cohort, the researchers applied AI to baseline MRI exams. They showed that patients not responding to chemotherapy were distinguished by “twisted” vessels, reduced structural organization, and reduced measures of vessel function.5 Yet other radiomic studies showed certain features to be associated with NSCLC’s response to immunotherapy and also the development of radiation-induced pneumonitis.
The ultimate proof of AI’s value will come from its prospective validation. To this end, Dr. Madabhushi’s group is partnering with the Eastern Cooperative Oncology Group “to demonstrate how we can start to embed these tools into clinical trials and start generating prospective data,” he said. In this way, “we can ensure we are developing affordable, accessible but also equitable AI approaches.”
DISCLOSURE: Dr. Madabhushi is a founder and equity partner for Picture Health. He reported financial or research-related relationships with Elucid Bioimaging, Inspirata, AstraZeneca, SimBioSys, Takeda, and Bristol Myers Squibb.
REFERENCES
1. Madabhushi A: Interpreter of maladies: Applications of AI for breast cancer prognosis and predicting treatment response. 2024 Miami Breast Cancer Conference. Scientific Keynote Address. Presented March 9, 2024.
2. Li H, Bera K, Toro P, et al: Collagen fiber orientation disorder from H&E images is prognostic for early stage breast cancer: Clinical trial validation. NPJ Breast Cancer 7:104, 2021.
3. Chen Y, Li H, Janowczyk A, et al: Computational pathology improves risk stratification of a multi-gene assay for early stage ER+ breast cancer. NPJ Breast Cancer 9:40, 2023.
4. Khorrami M, Viswanathan VS, Reddy P, et al: Radiomic predicts early response to CDK4/6 inhibitors in hormone receptor positive metastatic breast cancer. NPJ Breast Cancer 9:67, 2023.
5. Braman N, Prasanna P, Bera K, et al: Novel radiomic measurements of tumor-associated vasculature morphology on clinical imaging as a biomarker of treatment response in multiple cancers. Clin Cancer Res 28:4410-4424, 2022.