A blood-based, machine learning assay that combines cell-free DNA (cfDNA) fragmentomes and protein biomarkers was able to differentiate patients with ovarian cancer from healthy controls at a high specificity of more than 99%, according to study results by Medina et al.1 It noninvasively recognized benign masses from malignant ones, with an AUC of 0.87. The findings demonstrate the power of genome-wide cfDNA fragmentation and machine learning to detect ovarian cancer with high performance, potentially enabling a new paradigm for population-wide ovarian cancer screening and clinical diagnostic utility, according to the study authors. The study was presented during the American Association for Cancer Research (AACR) Annual Meeting 2024.1 The researchers intend to validate their models in larger cohorts of patients to strengthen the associations they observed in this study.
Ovarian cancer is the eighth most common cancer among women globally and the eighth most common cause of death from cancer worldwide.2 In the United States alone, in 2023, there were nearly 20,000 new cases of ovarian cancer and about 13,270 deaths from the disease.3 The reasons ovarian cancer is so deadly are because it is largely asymptomatic prior to metastasis, and there is no early cancer screening test.
Study Methodology
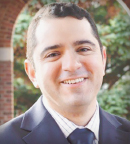
Jamie Medina, PhD
Liquid biopsy technologies have been explored as a way to noninvasively detect a variety of cancers; however, they have not always been useful in ovarian cancer, said Jamie Medina, PhD, a postdoctoral fellow at the Sidney Kimmel Comprehensive Cancer Center at Johns Hopkins Medicine and co–first author of this study, in a statement. He explained that DNA evaluation of fragments for early interception (DELFI) utilizes a newer method of liquid biopsy analysis, called fragmentomics, which has shown promise in improving the accuracy of such tests. The approach is based on detecting changes in the size and distribution of cfDNA fragments in the circulation across the genome, or the fragmentome.
The researchers developed a classifier using a combined fragmentomic-proteomic machine learning model incorporating cfDNA fragmentome using DELFI (DNA evaluation of fragments for early interception) and protein (CA-125 and HE4) features. This approach was evaluated for the detection of cancer in two clinical settings: screening for detection of cancers among a healthy population, and the diagnostic setting for classification of adnexal masses as benign or cancerous. They then assessed performance by cross-validation (n = 318 screening, n = 317 diagnostic) and externally validated the locked screening model using samples from a separate institution.
Key Results
The researchers found in the screening setting, the combined approach detected ovarian cancer with a specificity of more than 99% and a sensitivity of 69%, 76%, 85%, and 100% for stages I through IV, respectively (AUC = 0.97, 95% confidence interval [CI] = 0.94-0.99). At the same specificity, CA-125 alone detected 40%, 66%, 62%, and 100% of ovarian cancers for stages I through IV, highlighting the benefit of combining fragmentomic and proteomic features (P = .0009, two-sided test of equal proportions). Analysis of high-grade serous ovarian cancers (n = 10, 9, 24, 3 for stages I–IV, respectively) using their approach identified 91% of cases at a specific of more than 99%.
In an external set of cancer samples (n = 20), using the locked screening model and a fixed score threshold, the approach detected 65% of ovarian cancers. In the diagnostic setting, the approach differentiated benign masses from ovarian cancers with an AUC of 0.87 (95% CI = 0.83–0.91), detecting 60% of ovarian cancers at a specificity of 95%. In the preoperative setting, in which lower specificity may be acceptable, this approach may improve management of adnexal masses, according to the researchers.
“We demonstrate the utility of combining cell-free DNA fragmentomes and proteins for noninvasive detection of ovarian cancer. This approach detects ovarian cancers with high performance, potentially enabling a new paradigm for population-wide ovarian cancer screening and clinical diagnostic utility,” concluded the study authors.
Clinical Significance
“The lack of efficient screening tools, combined with the asymptomatic development of ovarian cancer, contributes to late diagnoses when effective treatment options are limited,” said Dr. Medina. “A cost-effective, accessible detection approach could change clinical paradigms of ovarian cancer screening and potentially save lives.”
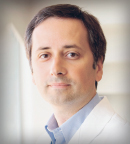
Victor Velculescu, MD, PhD, FAACR
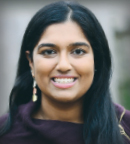
Akshaya Annapragada
“This study contributes to a large body of work from our group demonstrating the power of genome-wide cell-free DNA fragmentation and machine learning to detect cancers with high performance,” said Victor Velculescu, MD, PhD, FAACR, Professor of Oncology and Co-Director of the Cancer Genetics and Epigenetics Program at Johns Hopkins Kimmel Cancer Center, Baltimore, and senior author of the study. He added that “Our findings indicate that this combined approach resulted in improved performance for screening compared to existing biomarkers.”
Akshaya Annapragada, an MD/PhD student at the Johns Hopkins University School of Medicine, is a co–first author of this study.
DISCLOSURE: Dr. Medina reported no conflicts of interest. Dr. Velculescu has patent applications related to the use of cfDNA submitted by Johns Hopkins University and licensed to Delfi Diagnostics. For full disclosure information for the other study authors, visit abstractsonline.com.
REFERENCES
1. Medina JE, Annapragada AV, Lof P, et al: Early detection of ovarian cancer using cell-free DNA fragmentomes and protein biomarkers. 2024 AACR Annual Meeting. Abstract 6086. Presented April 9, 2024.
2. World Ovarian Cancer Coalition: Ovarian Cancer Key Stats. Available at https://worldovariancancercoalition.org/about-ovarian-cancer/key-stats. Accessed April 8. 2024.
3. National Cancer Institute: Cancer Stat Facts: Ovarian Cancer. Available at https://seer.cancer.gov/statfacts/html/ovary.html. Accessed April 8, 2024.