A machine learning model that uses a set or ensemble of algorithms has good accuracy for predicting colorectal cancer recurrence, investigators reported during a plenary session at the 2017 Society of Surgical Oncology (SSO) Annual Cancer Symposium.1
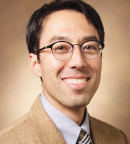
Multiple efforts to improve how we select patients [with colorectal cancer] for chemotherapy have been undertaken, including efforts to make molecular signatures, five of which have been commercialized. However, none of them are widely used in clinical practice.— Jason Castellanos, MD, MS
Tweet this quote
Persistent key questions in managing early colorectal cancer include which patients with stage II disease need adjuvant chemotherapy (as 20% will have a recurrence with resection alone) and which patients with stage III disease can forgo chemotherapy (as 50% will not have a recurrence if they skip it), noted first author Jason Castellanos, MD, MS, a resident in general surgery at Vanderbilt University Medical Center, Nashville.
“We are left with a treatment paradigm that both undertreats and overtreats certain populations,” he elaborated. “Multiple efforts to improve the way we select patients for chemotherapy have been undertaken, including efforts to make molecular signatures, five of which have been commercialized. However, none of them are widely used in clinical practice and none are U.S. Food and Drug Administration–approved for the task. That may be due to poor validation performance in original studies or poor generalizability on independent data sets.”
Complex Learning Model
The ensemble machine learning model that Dr. Castellanos and colleagues developed and validated uses complex methodology known as multiple-view, multiple-learner supervised learning.
Results derived with clinical and genomic data from 778 patients showed that the model had an AUC of 0.786 for predicting recurrence of stage II and III colorectal cancers, exceeding previously reported values for the ColoPrint, OncoDefender-CRC, and GeneFx Colon Risk Signature assays. Moreover, it outperformed the Oncotype DX Colon Recurrence Score for predicting 3-year disease-free survival.
“While the prediction accuracy of the model was better than the published and commercialized assays, a standard metric to be considered for something to be used for an individual patient’s prediction would be an AUC or F measure of 0.9. An AUC of 0.7 is a general cutoff for a population-based assay. So we are not quite there yet,” Dr. Castellanos commented.
The model is limited by its use of microarray data, now a somewhat outmoded platform, and the scant clinical data that were available in the databases used, he acknowledged.
Nonetheless, “we are hopeful that this will be a way to integrate different kinds of data types, such as multiomic data analysis, so we are actively looking into data sets that allow us to do that,” Dr. Castellanos concluded. “We are trying to look at more modern data sets, so we are excited that the American Association for Cancer Research has created the GENIE data set with more robust clinical information in a larger number of patients.”
Study Details
“An ensemble method combines multiple individual learning methods, and you usually get a better classification performance than you would with any single-base learning method,” Dr. Castellanos explained when introducing the study. “It’s an attractive and intuitive strategy, as it imitates our basic tendency to seek a variety of opinions when we make a major decision.”
For the study, the investigators tapped gene expression and clinical data from six publicly available microarray datasets. Analyses were based on data from 778 patients with stage II or III colorectal cancer: 624 in a training set and 154 in a validation set. Their respective rates of recurrence were 30% and 37%.
Results showed that a model using only the microarray data outperformed a model using basic clinical data for predicting recurrence, with an AUC of about 0.75 for the former and 0.65 for the latter (P < .001). “This suggests that microarray data can improve recurrence prediction above and beyond simple clinical data such as stage,” Dr. Castellanos noted.
Ensemble Learning to Predict Colorectal Cancer Recurrence
- In a study of 778 patients with stage II or III colorectal cancer, an ensemble machine learning model had an AUC of 0.786 for predicting recurrence.
- This was higher than AUCs reported for the ColoPrint (0.626), OncoDefender-CRC (0.55), and GeneFx Colon Risk Signature (0.684) assays.
- In the study’s validation cohort, Oncotype DX Colon Recurrence Score did not significantly predict 3-year disease-free survival, but the ensemble model did (P < .001).
In turn, models trained on three out of the four types of molecular views that the investigators evaluated—discretized, network expression mutation, and network—each outperformed the microarray data at this task, with AUCs of about 0.78 to 0.82 (P < .001).
The full ensemble prediction model had an AUC of 0.786 for discriminating patients who experienced recurrence, which was higher than the published validation AUCs for ColoPrint (0.626), OncoDefender-CRC (0.55), and GeneFx Colon Risk Signature (0.684). The model had a sensitivity of 0.947, a specificity of 0.649, a positive predictive value of 0.613, and a negative predictive value of 0.954.
Key Component
“We were curious as to what was doing the heavy lifting in this prediction framework,” Dr. Castellanos said. Analyses showed that, in particular, the network expression mutation component—a 547-gene signature capturing both genes previously identified in molecular signatures and tumor-suppressor and driver mutations—contributed about 30% of the model’s performance.
Finally, in the validation set, 3-year disease-free survival did not differ significantly between patients split by Oncotype DX into a low-risk group vs intermediate- or high-risk group, whereas patients split into predicted recurrence vs no recurrence groups by the ensemble model did (P < .001).
Findings were similar for patients with stage II disease and patients with stage III disease individually, according to Dr. Castellanos. ■
Disclosure: Dr. Castellanos reported no potential conflicts of interest.
Reference